This is an automated email from the ASF dual-hosted git repository. mayanks pushed a commit to branch dev in repository https://gitbox.apache.org/repos/asf/incubator-pinot-site.git
The following commit(s) were added to refs/heads/dev by this push: new 225a6f7 Moving to docusaurus style blogs pages new 1322024 Merge pull request #51 from ChethanUK/f/blog 225a6f7 is described below commit 225a6f7c0e8d98f29796d78818e618bcdbbf8adc Author: ChethanUK <chetha...@outlook.com> AuthorDate: Sun Jun 20 02:43:56 2021 +0530 Moving to docusaurus style blogs pages --- website/blog/2015-05-16-LinkedIn-Scaling.md | 24 ++++++++++++++++ website/blog/2017-09-17-Restaurant-Manager.md | 1 + ...Eye.md => 2019-01-09-LinkedIn-IntroThirdEye.md} | 2 +- ...StarTree.md => 2019-06-14-LinkedIn-StarTree.md} | 3 +- ...AutoTune.md => 2019-07-11-LinkedIn-AutoTune.md} | 1 + website/blog/2020-01-15-Pinot-Presto-SQL.md | 3 +- ...Thirdeye.md => 2020-02-20-LinkedIn-Thirdeye.md} | 6 ++-- .../blog/2020-04-10-DevBlog-AnalyzeGitEvents.md | 22 +++++++++++++++ ...lerts.md => 2020-06-25-LinkedIn-SmartAlerts.md} | 4 ++- ...ght.md => 2020-06-29-LinkedIn-TalentInsight.md} | 3 +- ...ime.md => 2020-07-14-LinkedIn-BatchRealtime.md} | 3 +- .../blog/2020-07-28-DevBlog-AnomalyDetection.md | 22 +++++++++++++++ website/blog/2020-07-28-DevBlog-DevUpStack.md | 20 ++++++++++++++ website/blog/2020-08-08-DevBlog-IngestPlugins.md | 32 ++++++++++++++++++++++ website/blog/2020-08-08-DevBlog-PinotMonitoring.md | 21 ++++++++++++++ website/blog/2020-08-08-DevBlog-SLAApps.md | 22 +++++++++++++++ website/blog/2020-08-08-DevBlog-ScalarUDFs.md | 26 ++++++++++++++++++ .../2020-10-16-DevBlog-TwitterTrollAnalysis.md | 23 ++++++++++++++++ website/blog/2021-01-08-DevBlog-DebeziumCDC.md | 23 ++++++++++++++++ website/blog/2021-02-02-DevBlog-PrestoPinot.md | 25 +++++++++++++++++ website/blog/2021-04-08-DevBlog-UpsertsIntro.md | 23 ++++++++++++++++ ...kedIn-Theta.md => 2021-04-16-LinkedIn-Theta.md} | 2 +- .../blog/2021-04-27-DevBlog-PinotInRetailChain.md | 21 ++++++++++++++ website/blog/2021-06-13-DevBlog-Geospatial.md | 23 ++++++++++++++++ website/blog/2021-06-16-LinkedIn-TextAnalytics.md | 22 +++++++++++++++ website/docusaurus.config.js | 4 +-- website/package.json | 12 ++++---- 27 files changed, 375 insertions(+), 18 deletions(-) diff --git a/website/blog/2015-05-16-LinkedIn-Scaling.md b/website/blog/2015-05-16-LinkedIn-Scaling.md new file mode 100644 index 0000000..b1e5d38 --- /dev/null +++ b/website/blog/2015-05-16-LinkedIn-Scaling.md @@ -0,0 +1,24 @@ +--- +title: A Brief History of Scaling LinkedIn +author: LinkedIn +author_title: LinkedIn Engineering Team +author_url: https://engineering.linkedin.com/blog/topic/pinot +author_image_url: https://upload.wikimedia.org/wikipedia/commons/thumb/e/e9/Linkedin_icon.svg/512px-Linkedin_icon.svg.png +description: + LinkedIn started in 2003 with the goal of connecting to your network for better job opportunities. It had only 2,700 members the first week. Fast forward many years, and LinkedIn’s product portfolio, member base, and server load has grown tremendously. +keywords: + - Pinot + - LinkedIn + - Data Scaling + - User-Facing Analytics + - Real-time data platform +tags: [Pinot, LinkedIn, real-time data platform, Realtime, Analytics, User-Facing Analytics] +--- + +LinkedIn started in 2003 with the goal of connecting to your network for better job opportunities. It had only 2,700 members the first week. Fast forward many years, and LinkedIn’s product portfolio, member base, and server load has grown tremendously. + +Today, LinkedIn operates globally with more than 350 million members. We serve tens of thousands of web pages every second of every day. We've hit our mobile moment where mobile accounts for more than 50 percent of all global traffic. All those requests are fetching data from our backend systems, which in turn handle millions of queries per second. + +Read More at https://engineering.linkedin.com/architecture/brief-history-scaling-linkedin + +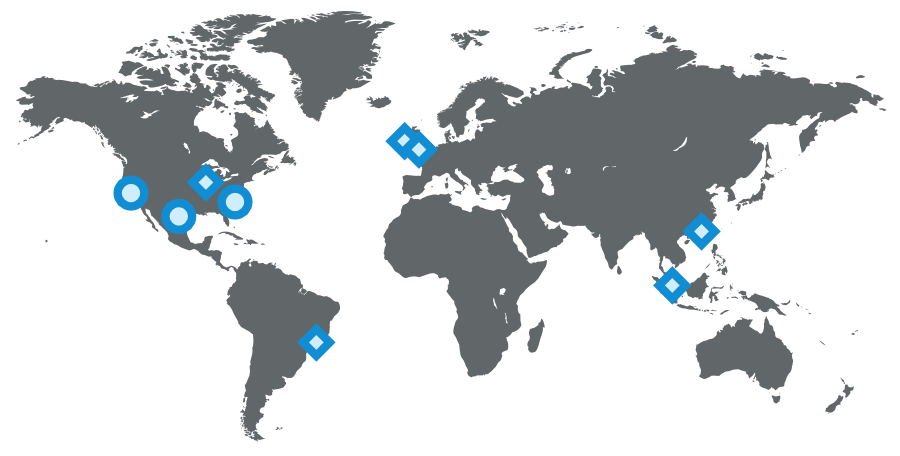 diff --git a/website/blog/2017-09-17-Restaurant-Manager.md b/website/blog/2017-09-17-Restaurant-Manager.md index bef49d4..bbb3c63 100644 --- a/website/blog/2017-09-17-Restaurant-Manager.md +++ b/website/blog/2017-09-17-Restaurant-Manager.md @@ -10,6 +10,7 @@ description: keywords: - Pinot - Uber Data + - User Analytics Dashboard - User-Facing Analytics - Real-time data platform tags: [Pinot, Uber Data, real-time data platform, Realtime, Analytics, User-Facing Analytics, financial intelligence] diff --git a/website/blog/2020-12-01-LinkedIn-IntroThirdEye.md b/website/blog/2019-01-09-LinkedIn-IntroThirdEye.md similarity index 98% rename from website/blog/2020-12-01-LinkedIn-IntroThirdEye.md rename to website/blog/2019-01-09-LinkedIn-IntroThirdEye.md index 1d249e0..d06b8e9 100644 --- a/website/blog/2020-12-01-LinkedIn-IntroThirdEye.md +++ b/website/blog/2019-01-09-LinkedIn-IntroThirdEye.md @@ -8,9 +8,9 @@ description: ThirdEye is a comprehensive platform for real-time monitoring of metrics that covers a wide variety of use-cases. keywords: - Pinot - - Uber Data - User-Facing Analytics - Real-time data platform + - ThirdEye tags: [Pinot, LinkedIn, real-time data platform, Realtime, ThirdEye, Analytics, User-Facing Analytics] --- diff --git a/website/blog/2020-12-01-LinkedIn-StarTree.md b/website/blog/2019-06-14-LinkedIn-StarTree.md similarity index 97% rename from website/blog/2020-12-01-LinkedIn-StarTree.md rename to website/blog/2019-06-14-LinkedIn-StarTree.md index 3554987..ba89022 100644 --- a/website/blog/2020-12-01-LinkedIn-StarTree.md +++ b/website/blog/2019-06-14-LinkedIn-StarTree.md @@ -9,7 +9,8 @@ description: while using the storage space efficiently. keywords: - Pinot - - Uber Data + - LinkedIn + - Star-Tree - User-Facing Analytics - Real-time data platform tags: [Pinot, LinkedIn, real-time data platform, Realtime, Analytics, User-Facing Analytics] diff --git a/website/blog/2020-12-01-LinkedIn-AutoTune.md b/website/blog/2019-07-11-LinkedIn-AutoTune.md similarity index 98% rename from website/blog/2020-12-01-LinkedIn-AutoTune.md rename to website/blog/2019-07-11-LinkedIn-AutoTune.md index bb10058..f860880 100644 --- a/website/blog/2020-12-01-LinkedIn-AutoTune.md +++ b/website/blog/2019-07-11-LinkedIn-AutoTune.md @@ -10,6 +10,7 @@ description: keywords: - Pinot - Uber Data + - Auto Tuning - User-Facing Analytics - Real-time data platform tags: [Pinot, LinkedIn, real-time data platform, Realtime, Analytics, User-Facing Analytics] diff --git a/website/blog/2020-01-15-Pinot-Presto-SQL.md b/website/blog/2020-01-15-Pinot-Presto-SQL.md index b6bf4ea..75ca1e5 100644 --- a/website/blog/2020-01-15-Pinot-Presto-SQL.md +++ b/website/blog/2020-01-15-Pinot-Presto-SQL.md @@ -11,7 +11,6 @@ keywords: - Pinot - Pinot SQL - Pinot Presto - - Uber Data - User-Facing Analytics - Real-time data platform tags: [Pinot, Uber, real-time data platform, Realtime, Analytics, User-Facing Analytics, Presto, SQL] @@ -21,6 +20,6 @@ Uber leverages real-time analytics on aggregate data to improve the user experie To resolve these issues, we built a solution that linked Presto, a query engine that supports full ANSI SQL, and Pinot, a real-time OLAP (online analytical processing) datastore. This married solution allows users to write ad-hoc SQL queries, empowering teams to unlock significant analysis capabilities. -Read More at https://eng.uber.com/engineering-sql-support-on-apache-pinot/ +[Read More at https://eng.uber.com/engineering-sql-support-on-apache-pinot/](https://eng.uber.com/engineering-sql-support-on-apache-pinot/)  diff --git a/website/blog/2020-12-01-LinkedIn-Thirdeye.md b/website/blog/2020-02-20-LinkedIn-Thirdeye.md similarity index 82% rename from website/blog/2020-12-01-LinkedIn-Thirdeye.md rename to website/blog/2020-02-20-LinkedIn-Thirdeye.md index 6258c9f..777d7d4 100644 --- a/website/blog/2020-12-01-LinkedIn-Thirdeye.md +++ b/website/blog/2020-02-20-LinkedIn-Thirdeye.md @@ -9,7 +9,9 @@ description: which allow us to efficiently figure out the unique size of a targeted audience when factoring in multiple criteria of an advertising campaign. keywords: - Pinot - - Uber Data + - LinkedIn + - Anomaly Detection + - Anomaly Alerts - User-Facing Analytics - Real-time data platform tags: [Pinot, LinkedIn, real-time data platform, Realtime, Analytics, User-Facing Analytics] @@ -18,6 +20,6 @@ tags: [Pinot, LinkedIn, real-time data platform, Realtime, Analytics, User-Facin Focus on the behind-the-scenes functionalities of ThirdEye that analyze the multi-dimensional time series data and help our engineers understand why these anomalies happened through a dimension heatmap -Read More at https://engineering.linkedin.com/blog/2020/analyzing-anomalies-with-thirdeye +[Read More at https://engineering.linkedin.com/blog/2020/analyzing-anomalies-with-thirdeye](https://engineering.linkedin.com/blog/2020/analyzing-anomalies-with-thirdeye) 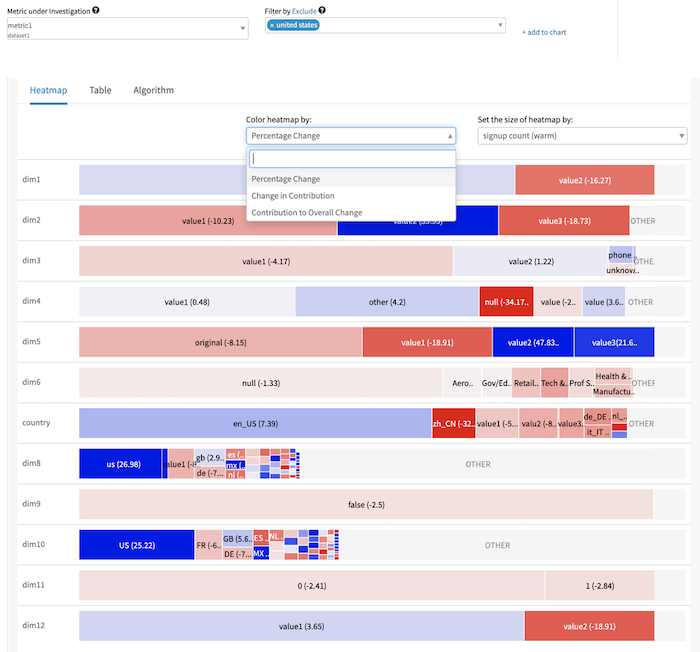 diff --git a/website/blog/2020-04-10-DevBlog-AnalyzeGitEvents.md b/website/blog/2020-04-10-DevBlog-AnalyzeGitEvents.md new file mode 100644 index 0000000..fc21fb4 --- /dev/null +++ b/website/blog/2020-04-10-DevBlog-AnalyzeGitEvents.md @@ -0,0 +1,22 @@ +--- +title: Using Apache Pinot and Kafka to Analyze GitHub Events +author: Kenny Bastani +author_title: Kenny Bastani +author_url: https://medium.com/apache-pinot-developer-blog +author_image_url: https://pbs.twimg.com/profile_images/1400521020973400069/5y2UMi4r_400x400.jpg +description: + Show you how Pinot and Kafka can be used together to ingest, query, and visualize event streams sourced from the public GitHub API. +keywords: + - Pinot + - LinkedIn + - User Analytics + - User-Facing Analytics + - Real-time data platform +tags: [Pinot, DevBlog, ThirdEye, real-time data platform, Realtime, Analytics, User-Facing Analytics] +--- + +In this blog post, we’ll show you how Pinot and Kafka can be used together to ingest, query, and visualize event streams sourced from the public GitHub API. For the step-by-step instructions, please visit our documentation, which will guide you through the specifics of running this example in your development environment. + +Read More at https://medium.com/apache-pinot-developer-blog/using-apache-pinot-and-kafka-to-analyze-github-events-93cdcb57d5f7 + + diff --git a/website/blog/2020-12-01-LinkedIn-SmartAlerts.md b/website/blog/2020-06-25-LinkedIn-SmartAlerts.md similarity index 95% rename from website/blog/2020-12-01-LinkedIn-SmartAlerts.md rename to website/blog/2020-06-25-LinkedIn-SmartAlerts.md index f844724..04c4e3c 100644 --- a/website/blog/2020-12-01-LinkedIn-SmartAlerts.md +++ b/website/blog/2020-06-25-LinkedIn-SmartAlerts.md @@ -9,8 +9,10 @@ description: which allow us to efficiently figure out the unique size of a targeted audience when factoring in multiple criteria of an advertising campaign. keywords: - Pinot - - Uber Data + - LinkedIn - User-Facing Analytics + - Smart alerts + - Automated User facing dashboards - Real-time data platform tags: [Pinot, LinkedIn, real-time data platform, Realtime, Analytics, User-Facing Analytics] --- diff --git a/website/blog/2020-12-01-LinkedIn-TalentInsight.md b/website/blog/2020-06-29-LinkedIn-TalentInsight.md similarity index 97% rename from website/blog/2020-12-01-LinkedIn-TalentInsight.md rename to website/blog/2020-06-29-LinkedIn-TalentInsight.md index a32f362..bcbed8f 100644 --- a/website/blog/2020-12-01-LinkedIn-TalentInsight.md +++ b/website/blog/2020-06-29-LinkedIn-TalentInsight.md @@ -9,7 +9,8 @@ description: which allow us to efficiently figure out the unique size of a targeted audience when factoring in multiple criteria of an advertising campaign. keywords: - Pinot - - Uber Data + - LinkedIn + - User Apps - User-Facing Analytics - Real-time data platform tags: [Pinot, LinkedIn, real-time data platform, Realtime, Analytics, User-Facing Analytics] diff --git a/website/blog/2020-12-01-LinkedIn-BatchRealtime.md b/website/blog/2020-07-14-LinkedIn-BatchRealtime.md similarity index 96% rename from website/blog/2020-12-01-LinkedIn-BatchRealtime.md rename to website/blog/2020-07-14-LinkedIn-BatchRealtime.md index 1c2350f..5a7d942 100644 --- a/website/blog/2020-12-01-LinkedIn-BatchRealtime.md +++ b/website/blog/2020-07-14-LinkedIn-BatchRealtime.md @@ -9,7 +9,8 @@ description: which allow us to efficiently figure out the unique size of a targeted audience when factoring in multiple criteria of an advertising campaign. keywords: - Pinot - - Uber Data + - LinkedIn + - Batch Realtime Data Pipelines - User-Facing Analytics - Real-time data platform tags: [Pinot, LinkedIn, real-time data platform, Realtime, Analytics, User-Facing Analytics] diff --git a/website/blog/2020-07-28-DevBlog-AnomalyDetection.md b/website/blog/2020-07-28-DevBlog-AnomalyDetection.md new file mode 100644 index 0000000..93628ba --- /dev/null +++ b/website/blog/2020-07-28-DevBlog-AnomalyDetection.md @@ -0,0 +1,22 @@ +--- +title: Building a culture around metrics and anomaly detection +author: Kenny Bastani +author_title: Kenny Bastani +author_url: https://medium.com/apache-pinot-developer-blog +author_image_url: https://pbs.twimg.com/profile_images/1400521020973400069/5y2UMi4r_400x400.jpg +description: + ThirdEye as a system is a platform that allows you to integrate your metrics (quantitative information) with events (knowledge or qualitative information) and combine the two so you can distinguish between meaningless anomalies and those ones that matter. +keywords: + - Pinot + - LinkedIn + - User-Facing Analytics + - Anomaly detection + - Real-time data platform +tags: [Pinot, DevBlog, ThirdEye, real-time data platform, Realtime, Analytics, User-Facing Analytics] +--- + +Anomaly detection is a very broad term. Usually it means that you want to see if things are running as usual. This could go from your business metrics down to the lowest level of how your systems are running. Anomaly detection is an entire process. It’s not just a tool that you get out of the box that measures time series data. Similar to DevOps, anomaly detection is a culture of different roles engaging in a process that combines tooling with human analysis. + +Read More at https://medium.com/apache-pinot-developer-blog/building-a-culture-around-metrics-and-anomaly-detection-da740960fcc2 + +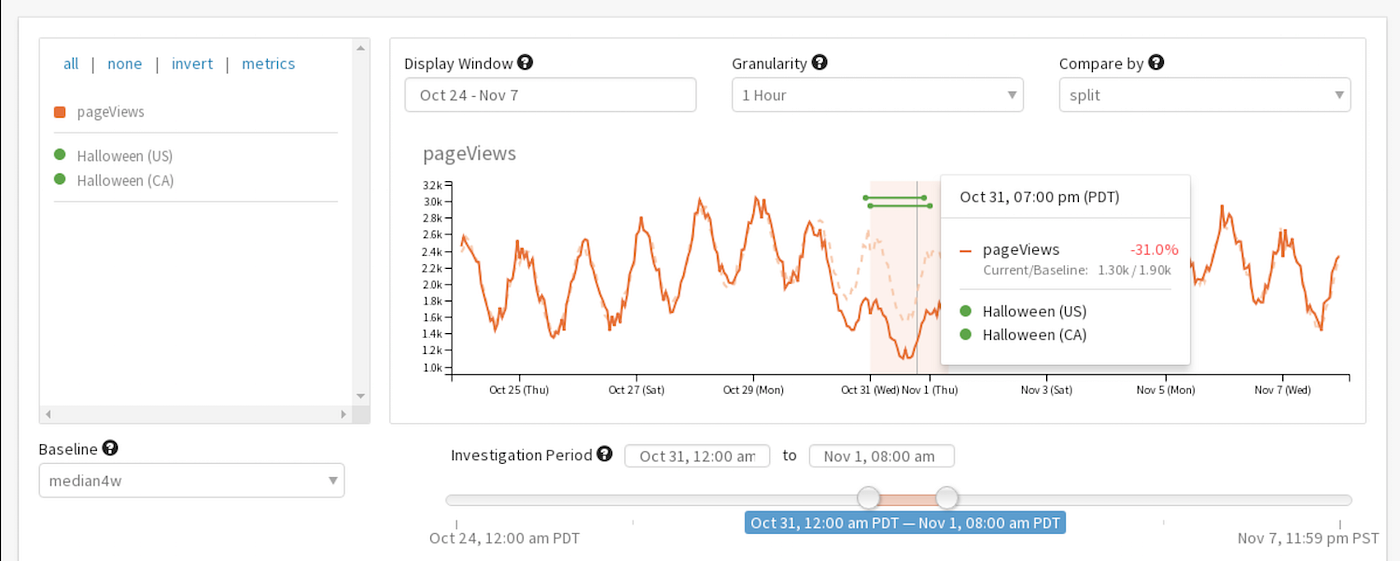 diff --git a/website/blog/2020-07-28-DevBlog-DevUpStack.md b/website/blog/2020-07-28-DevBlog-DevUpStack.md new file mode 100644 index 0000000..1e16d48 --- /dev/null +++ b/website/blog/2020-07-28-DevBlog-DevUpStack.md @@ -0,0 +1,20 @@ +--- +title: Moving developers up the stack with Apache Pinot +author: Kenny Bastani +author_title: Kenny Bastani +author_url: https://medium.com/apache-pinot-developer-blog +author_image_url: https://pbs.twimg.com/profile_images/1400521020973400069/5y2UMi4r_400x400.jpg +description: + Pinot enters into a storied legacy of innovations that have emerged from one of the world’s largest online social networks. Over a few decades, the Silicon Valley tech giant has helped hundreds of millions of people around the world navigate their careers. +keywords: + - Pinot + - User-Facing Analytics + - Real-time data platform +tags: [Pinot, DevBlog, ThirdEye, real-time data platform, Realtime, Analytics, User-Facing Analytics] +--- + +Once upon a time, an internet company named LinkedIn faced the challenge of having petabytes of connected data with no way to analyze it in real-time. As this was a problem that was the first of its kind, there was only one solution. The company put together a talented team of engineers and tasked them with building the right tool for the job. Today, that tool goes by the name of Apache Pinot. + +Read More at https://medium.com/apache-pinot-developer-blog/moving-developers-up-the-stack-with-apache-pinot-29d36717a3f4 + +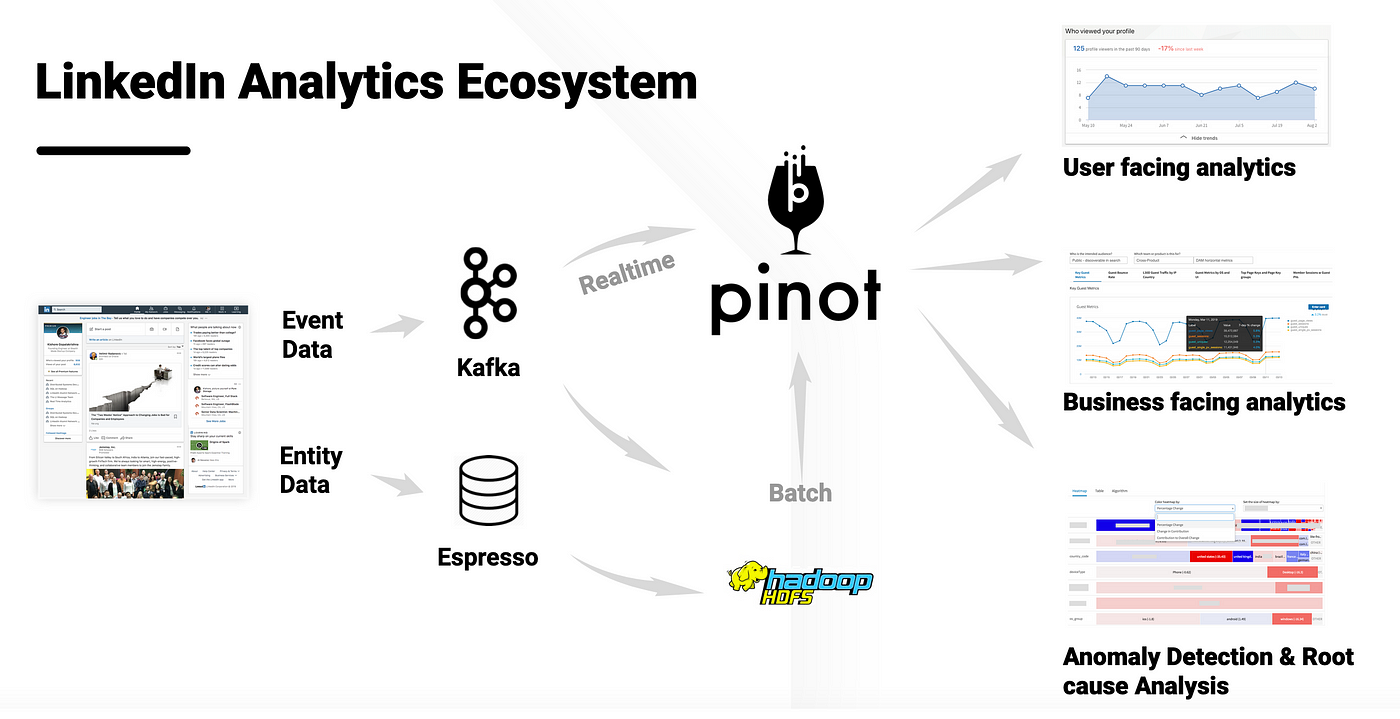 diff --git a/website/blog/2020-08-08-DevBlog-IngestPlugins.md b/website/blog/2020-08-08-DevBlog-IngestPlugins.md new file mode 100644 index 0000000..9b57907 --- /dev/null +++ b/website/blog/2020-08-08-DevBlog-IngestPlugins.md @@ -0,0 +1,32 @@ +--- +title: Leverage Plugins to Ingest Parquet Files from S3 in Pinot +author: PinotDev +author_title: Pinot Editorial Team +author_url: https://medium.com/apache-pinot-developer-blog +author_image_url: https://pbs.twimg.com/profile_images/1400521020973400069/5y2UMi4r_400x400.jpg +description: + Pinot is its pluggable architecture. The plugins make it easy to add support for any third-party system which can be an execution framework, a filesystem, or input format. +keywords: + - Pinot + - Plugins + - Ingestion + - Architecture + - Spark + - S3 + - Parquet + - User-Facing Analytics + - Real-time data platform +tags: [Pinot, SLA, DevBlog, ThirdEye, real-time data platform, Realtime, Analytics, User-Facing Analytics] +--- + +One of the primary advantages of using Pinot is its pluggable architecture. The plugins make it easy to add support for any third-party system which can be an execution framework, a filesystem, or input format. + +In this tutorial, we will use three such plugins to easily ingest data and push it to our Pinot cluster. The plugins we will be using are - + +- pinot-batch-ingestion-spark +- pinot-s3 +- pinot-parquet + +[Read more at https://medium.com/apache-pinot-developer-blog/leverage-plugins-to-ingest-parquet-files-from-s3-in-pinot-decb12e4d09d](https://medium.com/apache-pinot-developer-blog/leverage-plugins-to-ingest-parquet-files-from-s3-in-pinot-decb12e4d09d) + +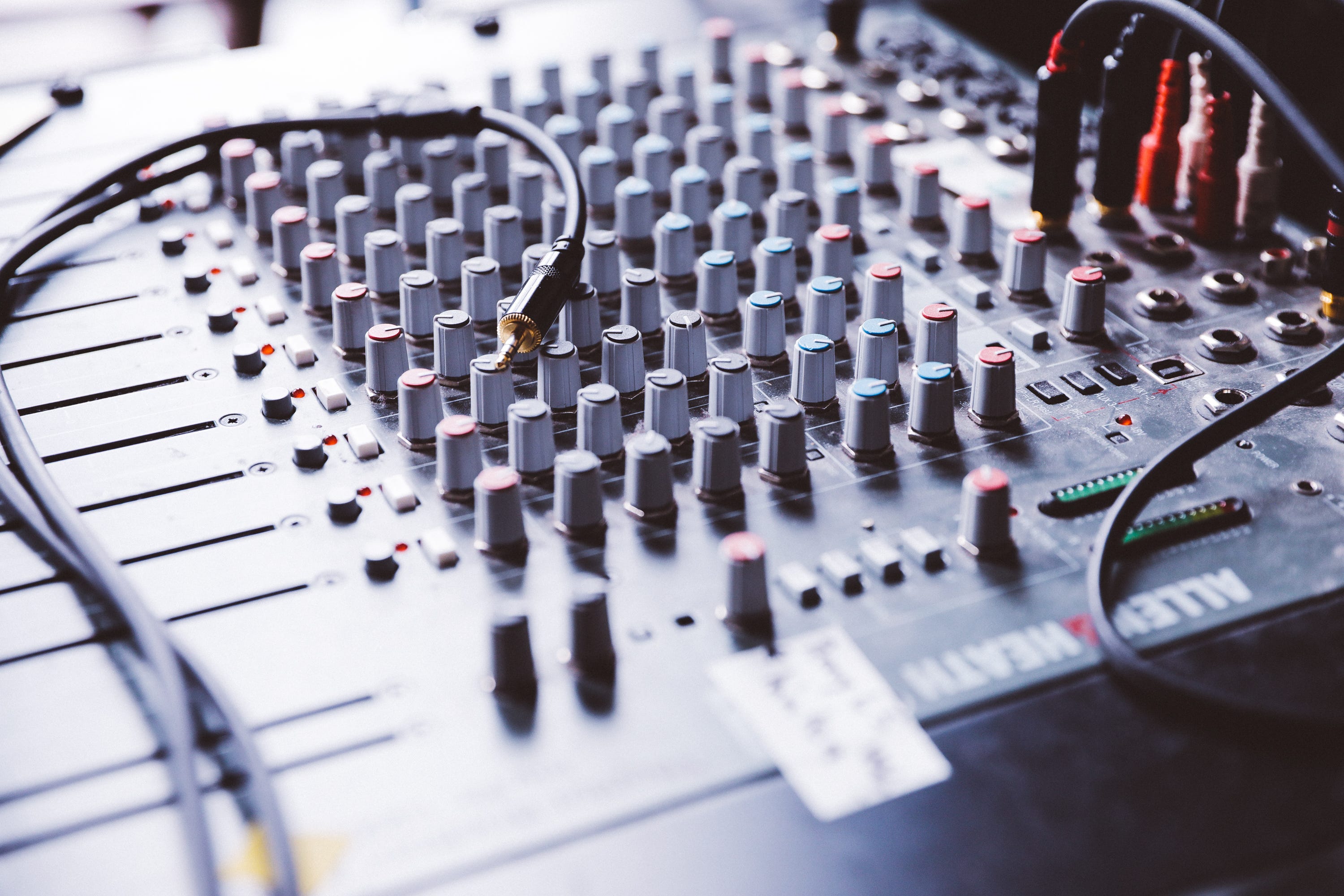 diff --git a/website/blog/2020-08-08-DevBlog-PinotMonitoring.md b/website/blog/2020-08-08-DevBlog-PinotMonitoring.md new file mode 100644 index 0000000..0f76401 --- /dev/null +++ b/website/blog/2020-08-08-DevBlog-PinotMonitoring.md @@ -0,0 +1,21 @@ +--- +title: Monitoring Apache Pinot with JMX, Prometheus and Grafana +author: PinotDev +author_title: Pinot Editorial Team +author_url: https://medium.com/apache-pinot-developer-blog +author_image_url: https://pbs.twimg.com/profile_images/1400521020973400069/5y2UMi4r_400x400.jpg +description: + Blog gives an overview of our use of Apache Pinot to solve some of biggest challenges around Data Analytics in Large Retail Chain +keywords: + - Pinot + - Monitoring + - User-Facing Analytics + - Real-time data platform +tags: [Pinot, Monitoring, JMX, Prometheus, Grafana, DevBlog, ThirdEye, real-time data platform, Realtime, Analytics, User-Facing Analytics] +--- + +I may be kicking open doors here, but a simple question has always helped me start from somewhere. When it comes to investigating degraded user experience caused by latency, can I observe high resource usage on all or some nodes of the system? + +[Read more at https://medium.com/apache-pinot-developer-blog/monitoring-apache-pinot-99034050c1a5](https://medium.com/apache-pinot-developer-blog/monitoring-apache-pinot-99034050c1a5) + +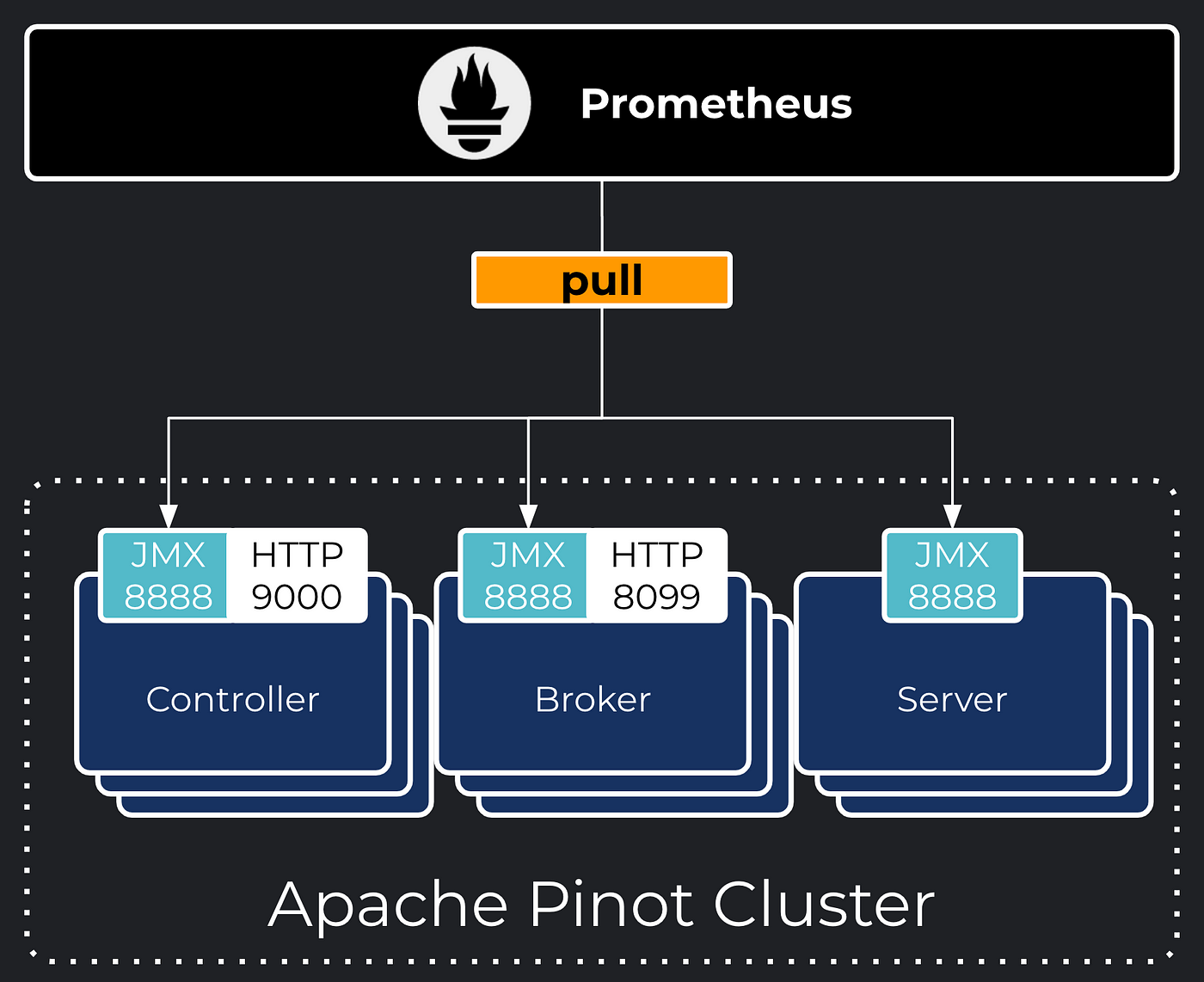 diff --git a/website/blog/2020-08-08-DevBlog-SLAApps.md b/website/blog/2020-08-08-DevBlog-SLAApps.md new file mode 100644 index 0000000..0249646 --- /dev/null +++ b/website/blog/2020-08-08-DevBlog-SLAApps.md @@ -0,0 +1,22 @@ +--- +title: Achieving 99th percentile latency SLA using Apache Pinot +author: PinotDev +author_title: Pinot Editorial Team +author_url: https://medium.com/apache-pinot-developer-blog +author_image_url: https://pbs.twimg.com/profile_images/1400521020973400069/5y2UMi4r_400x400.jpg +description: + How users can build critical site-facing analytical applications requiring high throughput and strict p99th query latency SLA +keywords: + - Pinot + - SLA + - User-Facing Apps + - User-Facing Analytics + - Real-time data platform +tags: [Pinot, SLA, DevBlog, ThirdEye, real-time data platform, Realtime, Analytics, User-Facing Analytics] +--- + +In this article, we talk about how users can build critical site-facing analytical applications requiring high throughput and strict p99th query latency SLA using Apache Pinot. + +[Read more at https://medium.com/apache-pinot-developer-blog/achieving-99th-percentile-latency-sla-using-apache-pinot-2ba4ce1d9eff](https://medium.com/apache-pinot-developer-blog/achieving-99th-percentile-latency-sla-using-apache-pinot-2ba4ce1d9eff) + +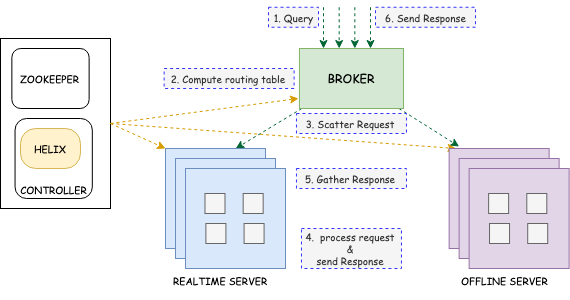 diff --git a/website/blog/2020-08-08-DevBlog-ScalarUDFs.md b/website/blog/2020-08-08-DevBlog-ScalarUDFs.md new file mode 100644 index 0000000..897d280 --- /dev/null +++ b/website/blog/2020-08-08-DevBlog-ScalarUDFs.md @@ -0,0 +1,26 @@ +--- +title: Utilize UDFs to Supercharge Queries in Apache Pinot +author: PinotDev +author_title: Pinot Editorial Team +author_url: https://medium.com/apache-pinot-developer-blog +author_image_url: https://pbs.twimg.com/profile_images/1400521020973400069/5y2UMi4r_400x400.jpg +description: + Scalar Functions that allow users to write and add their functions as a plugin. +keywords: + - Pinot + - UDF + - User-Facing Analytics + - Scalar Functions + - Real-time data platform +tags: [Pinot, SLA, DevBlog, ThirdEye, real-time data platform, Realtime, Analytics, User-Facing Analytics] +--- + +Apache Pinot is a realtime distributed OLAP datastore that can answer hundreds of thousands of queries with millisecond latencies. You can head over to https://pinot.apache.org/ to get started with Apache Pinot. + +While using any database, we can come across a scenario where a function required for the query is not supported out of the box. In such time, we have to resort to raising a pull request for a new function or finding a tedious workaround. + +Scalar Functions that allow users to write and add their functions as a plugin. + +[Read more at https://medium.com/apache-pinot-developer-blog/utilize-udfs-to-supercharge-queries-in-apache-pinot-e488a0f164f1](https://medium.com/apache-pinot-developer-blog/utilize-udfs-to-supercharge-queries-in-apache-pinot-e488a0f164f1) + +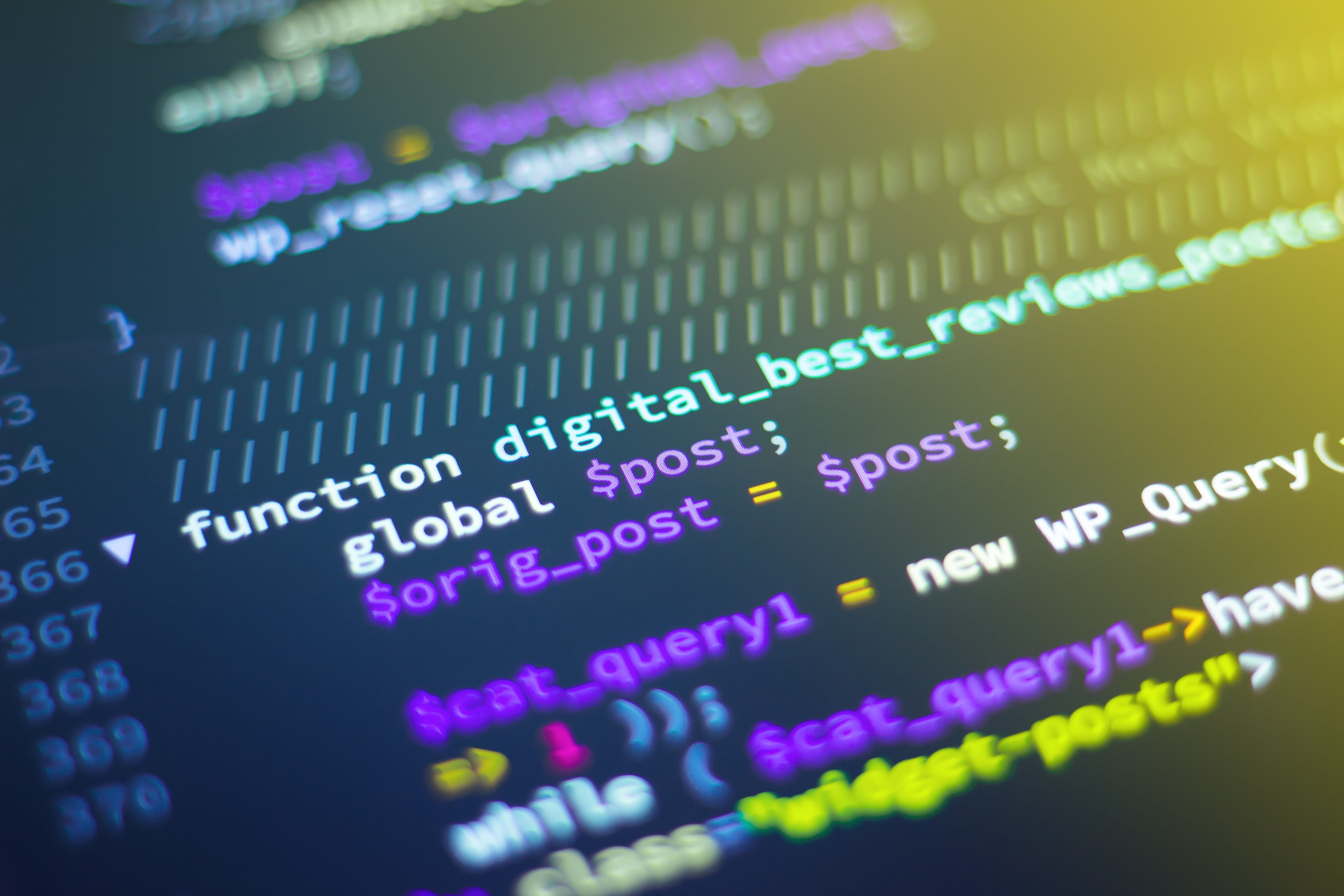 diff --git a/website/blog/2020-10-16-DevBlog-TwitterTrollAnalysis.md b/website/blog/2020-10-16-DevBlog-TwitterTrollAnalysis.md new file mode 100644 index 0000000..916b37c --- /dev/null +++ b/website/blog/2020-10-16-DevBlog-TwitterTrollAnalysis.md @@ -0,0 +1,23 @@ +--- +title: Deep Analysis of Russian Twitter Trolls +author: Kenny Bastani +author_title: Kenny Bastani +author_url: https://medium.com/apache-pinot-developer-blog +author_image_url: https://pbs.twimg.com/profile_images/1400521020973400069/5y2UMi4r_400x400.jpg +description: + Show you how to use Apache Pinot and Superset to analyze 3 million tweets by the Internet Research Agency (IRA) open-sourced by FiveThirtyEight. +keywords: + - Pinot + - Uber Data + - User-Facing Analytics + - Real-time data platform +tags: [Pinot, DevBlog, ThirdEye, real-time data platform, Realtime, Analytics, User-Facing Analytics] +--- + +The history behind Russian disinformation is a dense and continuously evolving subject. The world’s best research hasn’t seemed to hit the mainstream yet, which made this an excellent opportunity to see if I could use some open source tooling to surface new analytical evidence. + +In this blog post, I’ll show you how to use Apache Pinot and Superset to analyze 3 million tweets by the Internet Research Agency (IRA) open-sourced by FiveThirtyEight. + +[Read More at https://towardsdatascience.com/a-deep-analysis-of-russian-trolls-with-apache-pinot-and-superset-590c8c4d1843](https://towardsdatascience.com/a-deep-analysis-of-russian-trolls-with-apache-pinot-and-superset-590c8c4d1843) + +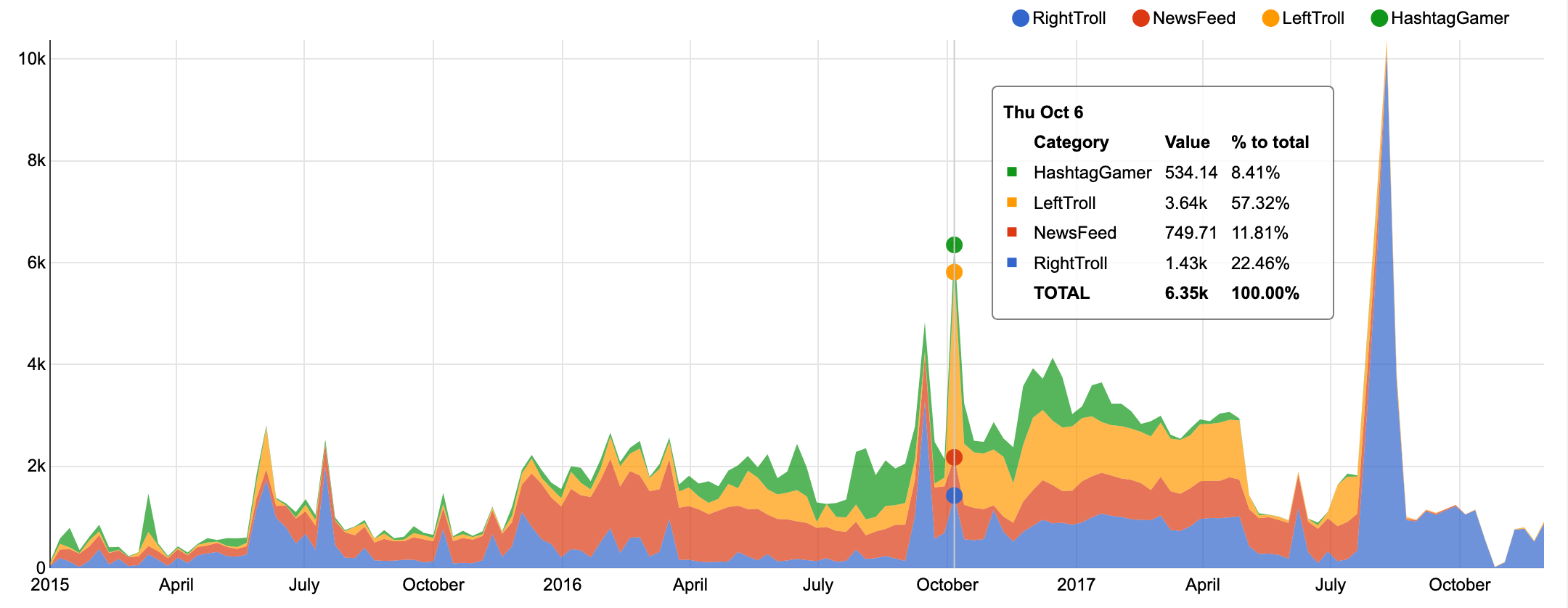 diff --git a/website/blog/2021-01-08-DevBlog-DebeziumCDC.md b/website/blog/2021-01-08-DevBlog-DebeziumCDC.md new file mode 100644 index 0000000..8307c79 --- /dev/null +++ b/website/blog/2021-01-08-DevBlog-DebeziumCDC.md @@ -0,0 +1,23 @@ +--- +title: Change Data Analysis with Debezium and Apache Pinot +author: Kenny Bastani +author_title: Kenny Bastani +author_url: https://medium.com/apache-pinot-developer-blog +author_image_url: https://pbs.twimg.com/profile_images/1400521020973400069/5y2UMi4r_400x400.jpg +description: + Pinot enters into a storied legacy of innovations that have emerged from one of the world’s largest online social networks. Over a few decades, the Silicon Valley tech giant has helped hundreds of millions of people around the world navigate their careers. +keywords: + - Pinot + - LinkedIn + - Change Data Capture Analytics + - User-Facing Analytics + - Debezium + - Real-time data platform +tags: [Pinot, DevBlog, Debezium, CDC, Change Data Analysis, real-time data platform, Realtime, Analytics, User-Facing Analytics] +--- + +In this blog post, we’re going to explore an exciting new world of real-time analytics based on combining the popular CDC tool, Debezium, with the real-time OLAP datastore, Apache Pinot. + +[Read More at https://medium.com/apache-pinot-developer-blog/change-data-analysis-with-debezium-and-apache-pinot-b4093dc178a7](https://medium.com/apache-pinot-developer-blog/change-data-analysis-with-debezium-and-apache-pinot-b4093dc178a7) + +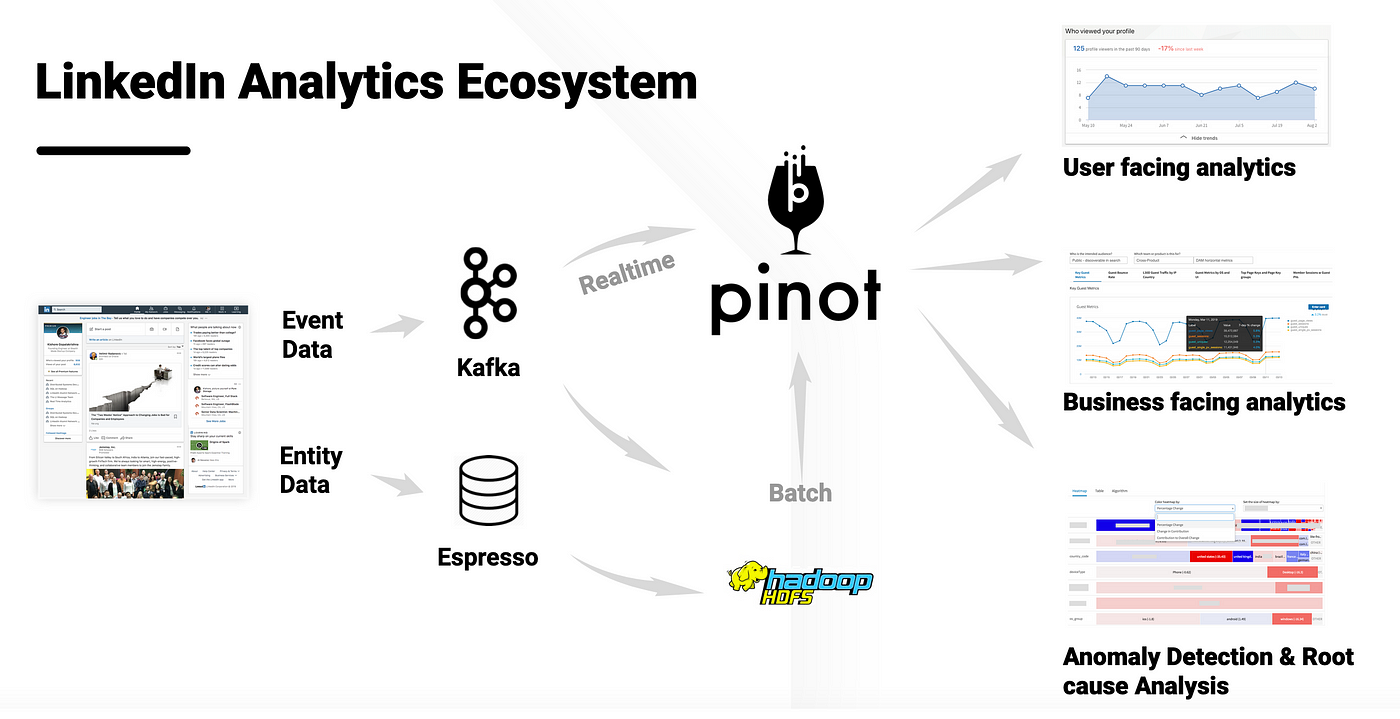 diff --git a/website/blog/2021-02-02-DevBlog-PrestoPinot.md b/website/blog/2021-02-02-DevBlog-PrestoPinot.md new file mode 100644 index 0000000..fb15ac0 --- /dev/null +++ b/website/blog/2021-02-02-DevBlog-PrestoPinot.md @@ -0,0 +1,25 @@ +--- +title: Real-time Analytics with Presto and Apache Pinot +author: PinotDev +author_title: Pinot Editorial Team +author_url: https://medium.com/apache-pinot-developer-blog +author_image_url: https://pbs.twimg.com/profile_images/1400521020973400069/5y2UMi4r_400x400.jpg +description: + Blog gives an overview of our use of Apache Pinot to solve some of biggest challenges around Data Analytics in Large Retail Chain +keywords: + - Pinot + - LinkedIn + - Trino + - Presto + - User-Facing Analytics + - Real-time data platform +tags: [Pinot, Presto, Trino, PrestoSQL, DevBlog, ThirdEye, real-time data platform, Realtime, Analytics, User-Facing Analytics] +--- + +In this world, most analytics products either focus on ad-hoc analytics, which requires query flexibility without guaranteed latency, or low latency analytics with limited query capability. In this blog, we will explore how to get the best of both worlds using Apache Pinot and Presto. + +[Read Part 1 at https://medium.com/apache-pinot-developer-blog/real-time-analytics-with-presto-and-apache-pinot-part-i-cc672caea307](https://medium.com/apache-pinot-developer-blog/real-time-analytics-with-presto-and-apache-pinot-part-i-cc672caea307) + +[Read Part 2 at https://medium.com/apache-pinot-developer-blog/real-time-analytics-with-presto-and-apache-pinot-part-ii-3d09ff937713](https://medium.com/apache-pinot-developer-blog/real-time-analytics-with-presto-and-apache-pinot-part-ii-3d09ff937713) + +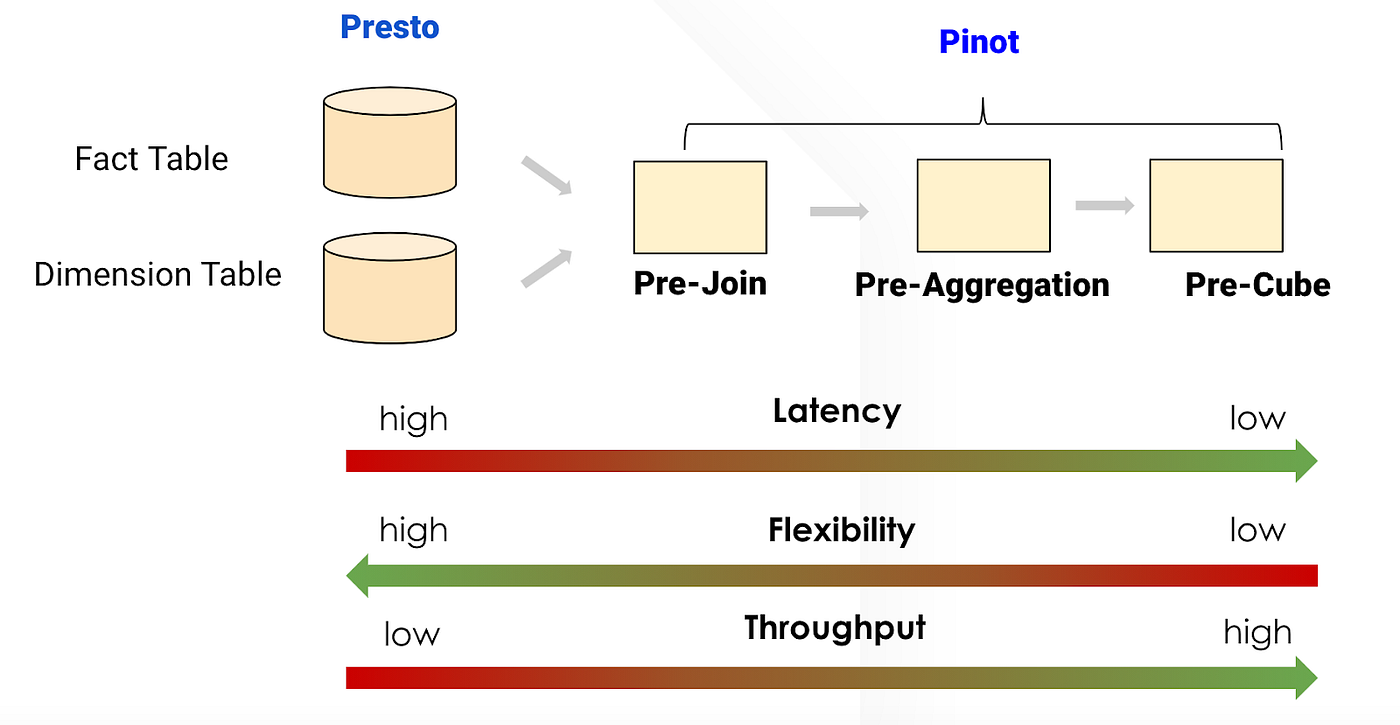 diff --git a/website/blog/2021-04-08-DevBlog-UpsertsIntro.md b/website/blog/2021-04-08-DevBlog-UpsertsIntro.md new file mode 100644 index 0000000..ab9f908 --- /dev/null +++ b/website/blog/2021-04-08-DevBlog-UpsertsIntro.md @@ -0,0 +1,23 @@ +--- +title: Introduction to Upserts in Apache Pinot +author: Kenny Bastani +author_title: Kenny Bastani +author_url: https://medium.com/apache-pinot-developer-blog +author_image_url: https://pbs.twimg.com/profile_images/1400521020973400069/5y2UMi4r_400x400.jpg +description: + Introduction to Pinot Upsert and explain why it’s exciting and how you can start using it. +keywords: + - Pinot + - Realtime Pipelines + - Upsert + - Kafka + - User-Facing Analytics + - Real-time data platform +tags: [Pinot, DevBlog, Upsert, real-time data platform, Realtime, Analytics, User-Facing Analytics] +--- + +Since the 0.6.0 release of Apache Pinot, a new feature was made available for stream ingestion that allows you to upsert events from an immutable log. Typically, upsert is a term used to describe inserting a record into a database if it does not already exist or update it if it does exist. In Apache Pinot’s case, upsert isn’t precisely the same concept, and I wanted to write this blog post to explain why it’s exciting and how you can start using it. + +Read More at https://medium.com/apache-pinot-developer-blog/introduction-to-upserts-in-apache-pinot-987c12149d93 + +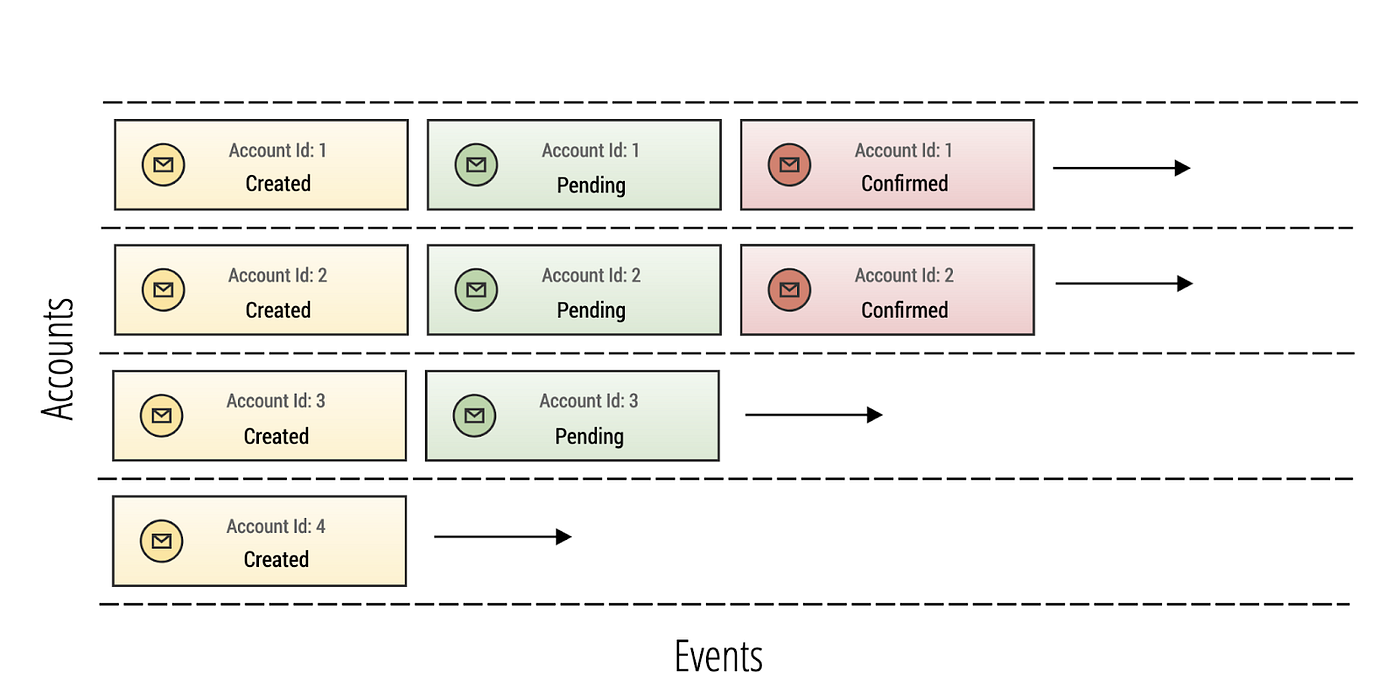 diff --git a/website/blog/2020-12-01-LinkedIn-Theta.md b/website/blog/2021-04-16-LinkedIn-Theta.md similarity index 98% rename from website/blog/2020-12-01-LinkedIn-Theta.md rename to website/blog/2021-04-16-LinkedIn-Theta.md index 9cf645a..b6cf7b3 100644 --- a/website/blog/2020-12-01-LinkedIn-Theta.md +++ b/website/blog/2021-04-16-LinkedIn-Theta.md @@ -9,7 +9,7 @@ description: which allow us to efficiently figure out the unique size of a targeted audience when factoring in multiple criteria of an advertising campaign. keywords: - Pinot - - Uber Data + - Theta Sketches - User-Facing Analytics - Real-time data platform tags: [Pinot, LinkedIn, real-time data platform, Realtime, Analytics, User-Facing Analytics] diff --git a/website/blog/2021-04-27-DevBlog-PinotInRetailChain.md b/website/blog/2021-04-27-DevBlog-PinotInRetailChain.md new file mode 100644 index 0000000..1a99af8 --- /dev/null +++ b/website/blog/2021-04-27-DevBlog-PinotInRetailChain.md @@ -0,0 +1,21 @@ +--- +title: Deploying Apache Pinot at a Large Retail Chain +author: PinotDev +author_title: Pinot Editorial Team +author_url: https://medium.com/apache-pinot-developer-blog +author_image_url: https://pbs.twimg.com/profile_images/1400521020973400069/5y2UMi4r_400x400.jpg +description: + Blog gives an overview of our use of Apache Pinot to solve some of biggest challenges around Data Analytics in Large Retail Chain +keywords: + - Pinot + - Retail Chain Use case + - User-Facing Analytics + - Real-time data platform +tags: [Pinot, DevBlog, ThirdEye, real-time data platform, Realtime, Analytics, User-Facing Analytics] +--- + +Blog gives an overview of our use of Apache Pinot to solve some of biggest challenges around Data Analytics in Large Retail Chain + +[Read More at https://medium.com/apache-pinot-developer-blog/deploying-apache-pinot-at-a-large-retail-chain-42aed2921a38](https://medium.com/apache-pinot-developer-blog/deploying-apache-pinot-at-a-large-retail-chain-42aed2921a38) + +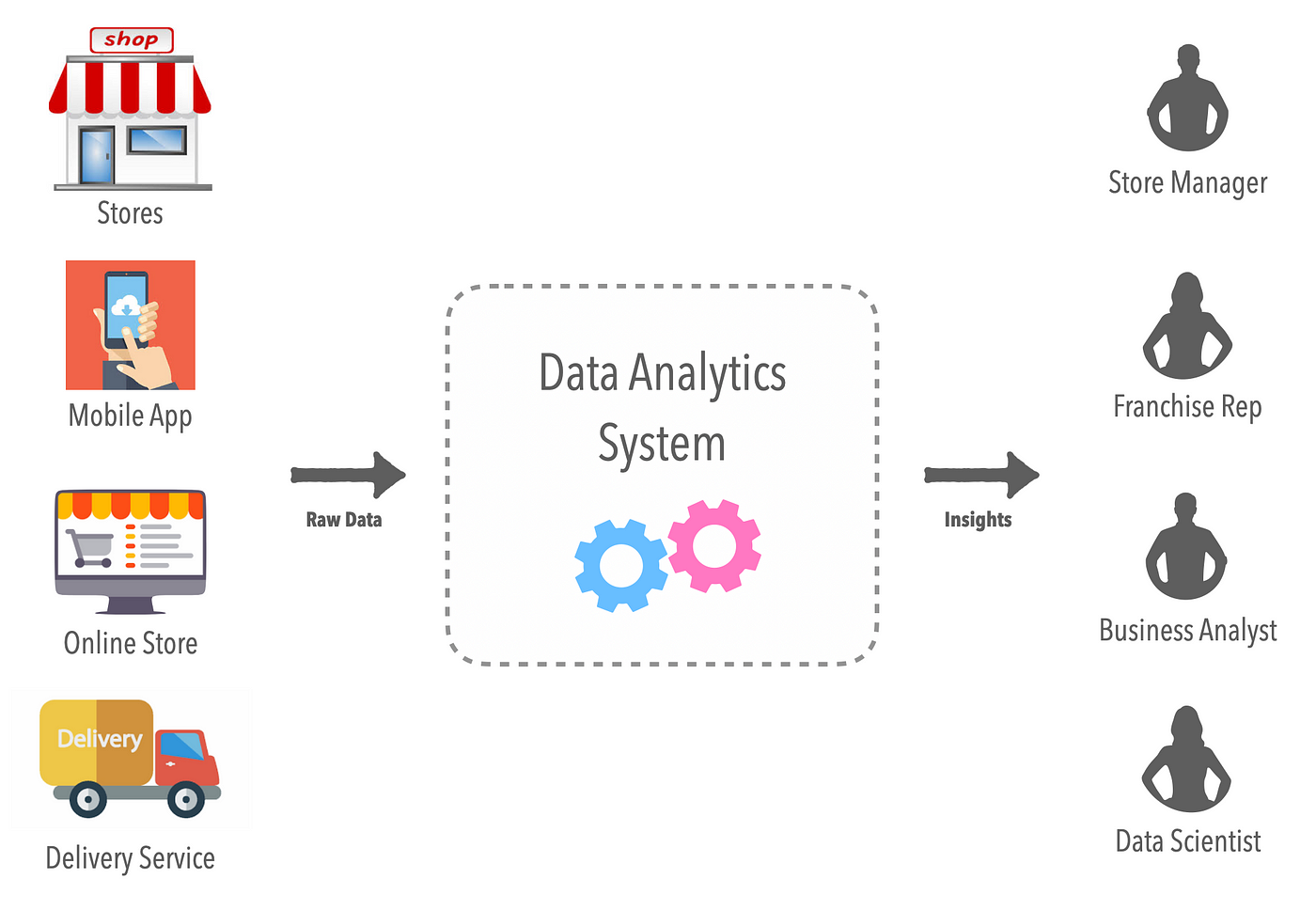 diff --git a/website/blog/2021-06-13-DevBlog-Geospatial.md b/website/blog/2021-06-13-DevBlog-Geospatial.md new file mode 100644 index 0000000..ac5a380 --- /dev/null +++ b/website/blog/2021-06-13-DevBlog-Geospatial.md @@ -0,0 +1,23 @@ +--- +title: Introduction to Geospatial Queries in Apache Pinot +author: Kenny Bastani +author_title: Kenny Bastani +author_url: https://medium.com/apache-pinot-developer-blog +author_image_url: https://pbs.twimg.com/profile_images/1400521020973400069/5y2UMi4r_400x400.jpg +description: + Discuss the challenges of analyzing geospatial at scale and propose the geospatial support in Pinot. +keywords: + - Pinot + - Geospatial + - H3 + - Index + - User-Facing Analytics + - Real-time data platform +tags: [Pinot, DevBlog, H3, real-time data platform, Realtime, Analytics, User-Facing Analytics] +--- + +Geospatial data has been widely used across the industry, spanning multiple verticals, such as ride-sharing and delivery, transportation infrastructure, defense and intel, public health. Deriving insights from timely and accurate geospatial data could enable mission-critical use cases in the organizations and fuel a vibrant marketplace across the industry. In the design document for this new Pinot feature, we discuss the challenges of analyzing geospatial at scale and propose the geospat [...] + +Read More at https://medium.com/apache-pinot-developer-blog/introduction-to-geospatial-queries-in-apache-pinot-b63e2362e2a9 + +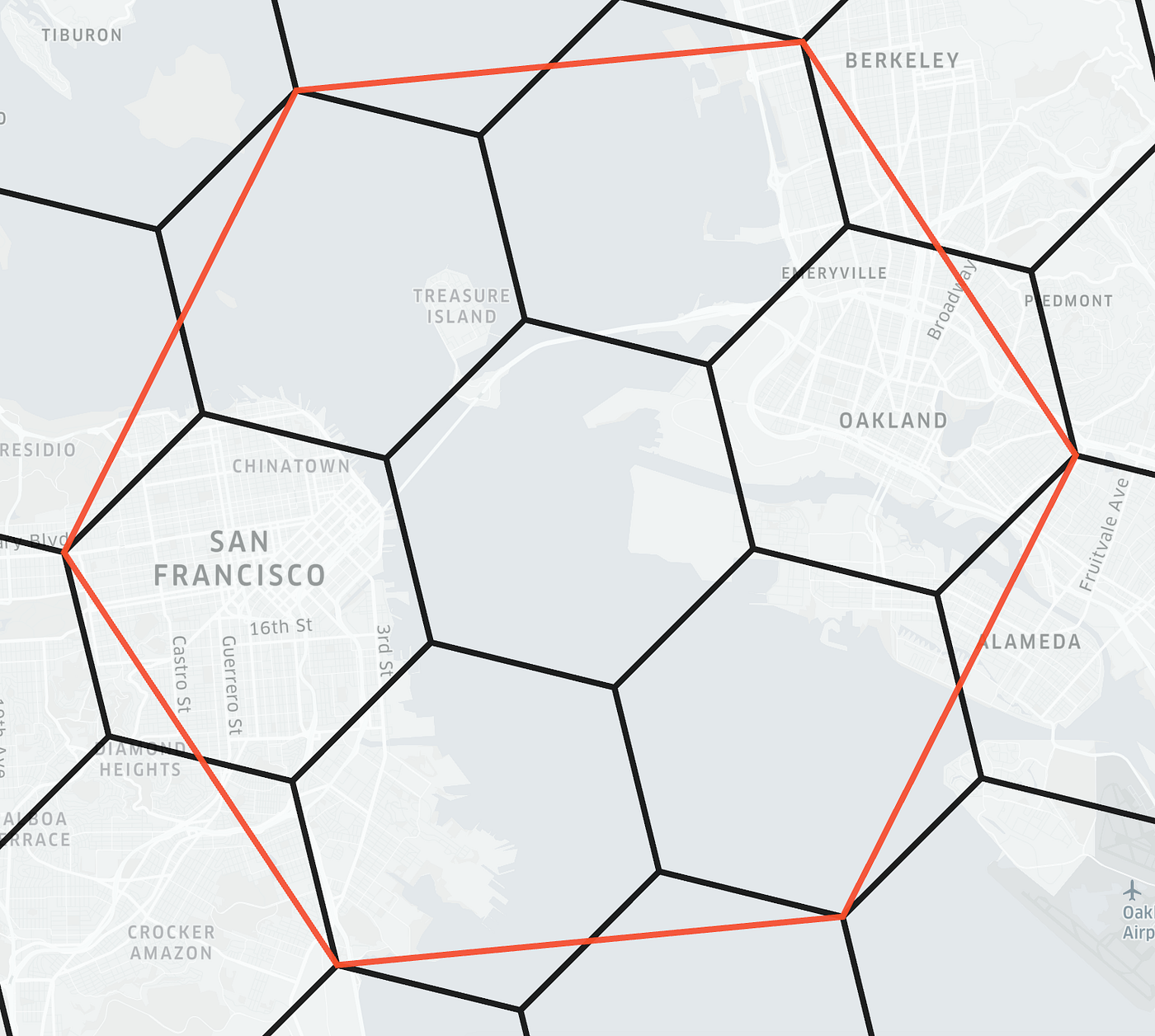 diff --git a/website/blog/2021-06-16-LinkedIn-TextAnalytics.md b/website/blog/2021-06-16-LinkedIn-TextAnalytics.md new file mode 100644 index 0000000..18265c1 --- /dev/null +++ b/website/blog/2021-06-16-LinkedIn-TextAnalytics.md @@ -0,0 +1,22 @@ +--- +title: Text analytics on LinkedIn Talent Insights using Apache Pinot +author: LinkedIn +author_title: LinkedIn Engineering Team +author_url: https://engineering.linkedin.com/blog/topic/pinot +author_image_url: https://upload.wikimedia.org/wikipedia/commons/thumb/e/e9/Linkedin_icon.svg/512px-Linkedin_icon.svg.png +description: + Introduction LinkedIn Talent Insights (LTI) is a platform that helps organizations understand the external labor market and their internal workforce, and enables the long term success of their employees +keywords: + - Pinot + - Text analytics + - Text index + - User-Facing Analytics + - Real-time data platform +tags: [Pinot, LinkedIn, Data, Text analytics, real-time data platform, Realtime, ThirdEye, Analytics, User-Facing Analytics] +--- + +LinkedIn Talent Insights (LTI) is a platform that helps organizations understand the external labor market and their internal workforce, and enables the long term success of their employees. Users of LTI have the flexibility to construct searches using the various facets of the LinkedIn Economic Graph (skills, titles, location, company, etc.). + +[Read More at https://engineering.linkedin.com/blog/2021/text-analytics-on-linkedin-talent-insights-using-apache-pinot](https://engineering.linkedin.com/blog/2021/text-analytics-on-linkedin-talent-insights-using-apache-pinot) + +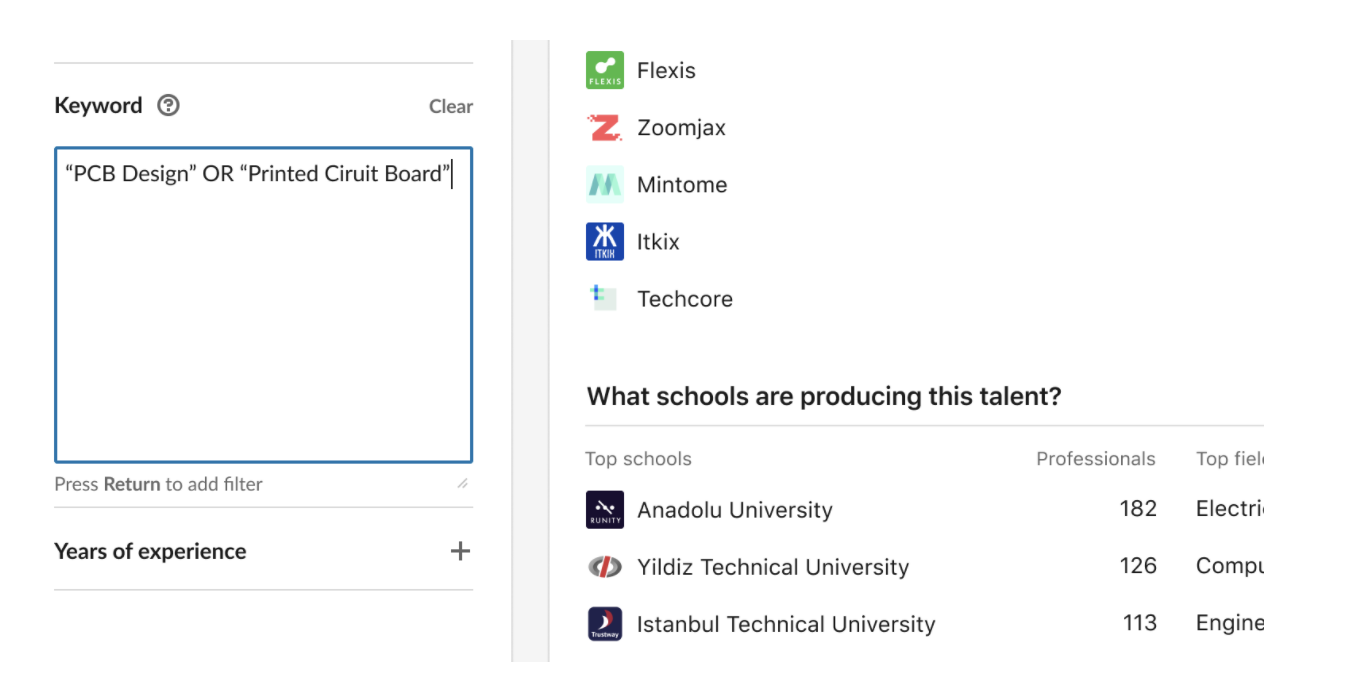 diff --git a/website/docusaurus.config.js b/website/docusaurus.config.js index 6bfbac4..3600906 100755 --- a/website/docusaurus.config.js +++ b/website/docusaurus.config.js @@ -45,8 +45,8 @@ module.exports = { items: [ {to: 'https://docs.pinot.apache.org/', label: 'Docs', position: 'right'}, {to: '/download', label: 'Download', position: 'right'}, - // {to: '/blog', label: 'Blog', position: 'right'}, - {to: 'https://docs.pinot.apache.org/community-1/blogs', label: 'Blog', position: 'right'}, + {to: '/blog', label: 'Blog', position: 'right'}, + // {to: 'https://docs.pinot.apache.org/community-1/blogs', label: 'Blog', position: 'right'}, { href: 'https://github.com/apache/incubator-pinot', label: 'GitHub', diff --git a/website/package.json b/website/package.json index 0b55325..3391d89 100755 --- a/website/package.json +++ b/website/package.json @@ -16,11 +16,11 @@ "write-heading-ids": "docusaurus write-heading-ids" }, "dependencies": { - "@docusaurus/core": "2.0.0-beta.0", - "@docusaurus/plugin-content-blog": "2.0.0-beta.0", - "@docusaurus/plugin-ideal-image": "2.0.0-beta.0", - "@docusaurus/preset-classic": "2.0.0-beta.0", - "@docusaurus/utils": "2.0.0-beta.0", + "@docusaurus/core": "^2.0.0-beta.1", + "@docusaurus/plugin-content-blog": "^2.0.0-beta.1", + "@docusaurus/plugin-ideal-image": "^2.0.0-beta.1", + "@docusaurus/preset-classic": "^2.0.0-beta.1", + "@docusaurus/utils": "^2.0.0-beta.1", "@farbenmeer/react-spring-slider": "^1.3.4", "axios": "^0.21.1", "cash-dom": "^8.1.0", @@ -46,7 +46,7 @@ "svg.js": "^2.7.1" }, "devDependencies": { - "@docusaurus/module-type-aliases": "2.0.0-beta.0", + "@docusaurus/module-type-aliases": "^2.0.0-beta.1", "@tsconfig/docusaurus": "^1.0.2", "@types/react": "^17.0.3", "@types/react-helmet": "^6.1.1", --------------------------------------------------------------------- To unsubscribe, e-mail: commits-unsubscr...@pinot.apache.org For additional commands, e-mail: commits-h...@pinot.apache.org