This is an automated email from the ASF dual-hosted git repository. luzhijing pushed a commit to branch master in repository https://gitbox.apache.org/repos/asf/doris-website.git
The following commit(s) were added to refs/heads/master by this push: new 9ceeed4ddda Add blog-inverted index (#234) 9ceeed4ddda is described below commit 9ceeed4dddaf093badaa0cca610719eda28039eb Author: Hu Yanjun <100749531+httpshir...@users.noreply.github.com> AuthorDate: Fri May 26 22:28:33 2023 +0800 Add blog-inverted index (#234) --- blog/Inverted Index.md | 249 ++++++++++++++++++ .../Inverted Index.md | 281 +++++++++++++++++++++ static/images/Inverted_1.png | Bin 0 -> 110644 bytes static/images/Inverted_2.png | Bin 0 -> 457762 bytes static/images/Inverted_3.png | Bin 0 -> 61226 bytes static/images/Inverted_4.png | Bin 0 -> 192833 bytes static/images/Inverted_5.png | Bin 0 -> 157152 bytes static/images/Inverted_6.png | Bin 0 -> 137468 bytes 8 files changed, 530 insertions(+) diff --git a/blog/Inverted Index.md b/blog/Inverted Index.md new file mode 100644 index 00000000000..a3286745161 --- /dev/null +++ b/blog/Inverted Index.md @@ -0,0 +1,249 @@ +--- +{ + 'title': 'Building A Log Analytics Solution 10 Times More Cost-Effective Than Elasticsearch', + 'summary': "Apache Doris has introduced inverted indexes in version 2.0.0 and further optimized it to realize two times faster log query performance than Elasticsearch with 1/5 of the storage space it uses.", + 'date': '2023-05-26', + 'author': 'Apache Doris', + 'tags': ['Tech Sharing'], +} + +--- + +<!-- +Licensed to the Apache Software Foundation (ASF) under one +or more contributor license agreements. See the NOTICE file +distributed with this work for additional information +regarding copyright ownership. The ASF licenses this file +to you under the Apache License, Version 2.0 (the +"License"); you may not use this file except in compliance +with the License. You may obtain a copy of the License at + + http://www.apache.org/licenses/LICENSE-2.0 + +Unless required by applicable law or agreed to in writing, +software distributed under the License is distributed on an +"AS IS" BASIS, WITHOUT WARRANTIES OR CONDITIONS OF ANY +KIND, either express or implied. See the License for the +specific language governing permissions and limitations +under the License. +--> + + + +Logs often take up the majority of a company's data assets. Examples of logs include business logs (such as user activity logs), and Operation & Maintenance logs of servers, databases, and network or IoT devices. + +Logs are the guardian angel of business. On the one hand, they provide system risk alerts and help engineers in troubleshooting. On the other hand, if you zoom them out by time range, you might identify some helpful trends and patterns, not to mention that business logs are the cornerstone of user insights. + +However, logs can be a handful, because: + +- **They flow in like crazy.** Every system event or click from user generates a log. A company often produces tens of billions of new logs per day. +- **They are bulky.** Logs are supposed to stay. They might not be useful until they are. So a company can accumulate up to PBs of log data, many of which are seldom visited but take up huge storage space. +- **They must be quick to load and find.** Locating the target log for troubleshooting is literally like looking for a needle in a haystack. People long for real-time log writing and real-time responses to log queries. + +Now you can see a clear picture of what an ideal log processing system is like. It should support: + +- **High-throughput real-time data ingestion:** It should be able to write blogs in bulk, and make them visible immediately. +- **Low-cost storage:** It should be able to store substantial amounts of logs without costing too many resources. +- **Real-time text search:** It should be capable of quick text search. + +## Common Solutions: Elasticsearch & Grafana Loki + +There exist two common log processing solutions within the industry, exemplified by Elasticsearch and Grafana Loki, respectively. + +- **Inverted index (Elasticsearch)**: It is well-embraced due to its support for full-text search and high performance. The downside is the low throughput in real-time writing and the huge resource consumption in index creation. +- **Lightweight index / no index (Grafana Loki)**: It is the opposite of inverted index because it boasts high real-time write throughput and low storage cost but delivers slow queries. + + + +## Introduction to Inverted Index + +A prominent strength of Elasticsearch in log processing is quick keyword search among a sea of logs. This is enabled by inverted indexes. + +Inverted indexing was originally used to retrieve words or phrases in texts. The figure below illustrates how it works: + +Upon data writing, the system tokenizes texts into **terms**, and stores these terms in a **posting list** which maps terms to the ID of the row where they exist. In text queries, the database finds the corresponding **row ID** of the keyword (term) in the posting list, and fetches the target row based on the row ID. By doing so, the system won't have to traverse the whole dataset and thus improves query speeds by orders of magnitudes. + + + +In inverted indexing of Elasticsearch, quick retrieval comes at the cost of writing speed, writing throughput, and storage space. Why? Firstly, tokenization, dictionary sorting, and inverted index creation are all CPU- and memory-intensive operations. Secondly, Elasticssearch has to store the original data, the inverted index, and an extra copy of data stored in columns for query acceleration. That's triple redundancy. + +But without inverted index, Grafana Loki, for example, is hurting user experience with its slow queries, which is the biggest pain point for engineers in log analysis. + +Simply put, Elasticsearch and Grafana Loki represent different tradeoffs between high writing throughput, low storage cost, and fast query performance. What if I tell you there is a way to have them all? We have introduced inverted indexes in [Apache Doris 2.0.0](https://github.com/apache/doris/issues/19231) and further optimized it to realize **two times faster log query performance than Elasticsearch with 1/5 of the storage space it uses. Both factors combined, it is a 10 times better [...] + +## Inverted Index in Apache Doris + +Generally, there are two ways to implement indexes: **external indexing system** or **built-in indexes**. + +**External indexing system:** You connect an external indexing system to your database. In data ingestion, data is imported to both systems. After the indexing system creates indexes, it deletes the original data within itself. When data users input a query, the indexing system provides the IDs of the relevant data, and then the database looks up the target data based on the IDs. + +Building an external indexing system is easier and less intrusive to the database, but it comes with some annoying flaws: + +- The need to write data into two systems can result in data inconsistency and storage redundancy. +- Interaction between the database and the indexing system brings overheads, so when the target data is huge, the query across the two systems can be slow. +- It is exhausting to maintain two systems. + +In [Apache Doris](https://github.com/apache/doris), we opt for the other way. Built-in inverted indexes are more difficult to make, but once it is done, it is faster, more user-friendly, and trouble-free to maintain. + +In Apache Doris, data is arranged in the following format. Indexes are stored in the Index Region: + + + +We implement inverted indexes in a non-intrusive manner: + +1. **Data ingestion & compaction**: As a segment file is written into Doris, an inverted index file will be written, too. The index file path is determined by the segment ID and the index ID. Rows in segments correspond to the docs in indexes, so are the RowID and the DocID. +2. **Query**: If the `where` clause includes a column with inverted index, the system will look up in the index file, return a DocID list, and convert the DocID list into a RowID Bitmap. Under the RowID filtering mechanism of Apache Doris, only the target rows will be read. This is how queries are accelerated. + + + +Such non-intrusive method separates the index file from the data files, so you can make any changes to the inverted indexes without worrying about affecting the data files themselves or other indexes. + +## Optimizations for Inverted Index + +### General Optimizations + +**C++ Implementation and Vectorization** + +Different from Elasticsearch, which uses Java, Apache Doris implements C++ in its storage modules, query execution engine, and inverted indexes. Compared to Java, C++ provides better performance, allows easier vectorization, and produces no JVM GC overheads. We have vectorized every step of inverted indexing in Apache Doris, such as tokenization, index creation, and queries. To provide you with a perspective, **in inverted indexing, Apache Doris writes data at a speed of 20MB/s per core, [...] + +**Columnar Storage & Compression** + +Apache Lucene lays the foundation for inverted indexes in Elasticsearch. As Lucene itself is built to support file storage, it stores data in a row-oriented format. + +In Apache Doris, inverted indexes for different columns are isolated from each other, and the inverted index files adopt columnar storage to facilitate vectorization and data compression. + +By utilizing Zstandard compression, Apache Doris realizes a compression ratio ranging from **5:1** to **10:1**, faster compression speeds, and 50% less space usage than GZIP compression. + +**BKD Trees for Numeric / Datetime Columns** + +Apache Doris implements BKD trees for numeric and datetime columns. This not only increases performance of range queries, but is a more space-saving method than converting those columns to fixed-length strings. Other benefits of it include: + +1. **Efficient range queries**: It is able to quickly locate the target data range in numeric and datetime columns. +2. **Less storage space**: It aggregates and compresses adjacent data blocks to reduce storage costs. +3. **Support for multi-dimensional data**: BKD trees are scalable and adaptive to multi-dimensional data types, such as GEO points and ranges. + +In addition to BKD trees, we have further optimized the queries on numeric and datetime columns. + +1. **Optimization for low-cardinality scenarios**: We have fine-tuned the compression algorithm for low-cardinality scenarios, so decompressing and de-serializing large amounts of inverted lists will consume less CPU resources. +2. **Pre-fetching**: For high-hit-rate scenarios, we adopt pre-fetching. If the hit rate exceeds a certain threshold, Doris will skip the indexing process and start data filtering. + +### Tailored Optimizations to OLAP + +Log analysis is a simple kind of query with no need for advanced features (e.g. relevance scoring in Apache Lucene). The bread and butter capability of a log processing tool is quick queries and low storage cost. Therefore, in Apache Doris, we have streamlined the inverted index structure to meet the needs of an OLAP database. + +- In data ingestion, we prevent multiple threads from writing data into the same index, and thus avoid overheads brought by lock contention. +- We discard forward index files and Norm files to clear storage space and reduce I/O overheads. +- We simplify the computation logic of relevance scoring and ranking to further reduce overheads and increase performance. + +In light of the fact that logs are partitioned by time range and historical logs are visited less frequently. We plan to provide more granular and flexible index management in future versions of Apache Doris: + +- **Create inverted index for a specified data partition**: create index for logs of the past seven days, etc. +- **Delete** **inverted index for a specified data partition**: delete index for logs from over one month ago, etc. (so as to clear out index space). + +## Benchmarking + +We tested Apache Doris on publicly available datasets against Elasticsearch and ClickHouse. + +For a fair comparison, we ensure uniformity of testing conditions, including benchmarking tool, dataset, and hardware. + +### Apache Doris VS Elasticsearch + +**Benchmarking tool**: ES Rally, the official testing tool for Elasticsearch + +**Dataset**: 1998 World Cup HTTP Server Logs (self-contained dataset in ES Rally) + +**Data Size (Before Compression)**: 32G, 247 million rows, 134 bytes per row (on average) + +**Query**: 11 queries including keyword search, range query, aggregation, and ranking; Each query is serially executed 100 times. + +**Environment**: 3 × 16C 64G cloud virtual machines + +- **Results of Apache Doris**: + +- - Writing Speed: 550 MB/s, **4.2 times that of Elasticsearch** + - Compression Ratio: 10:1 + - Storage Usage: **20% that of Elasticsearch** + - Response Time: **43% that of Elasticsearch** + + + +### Apache Doris VS ClickHouse + +As ClickHouse launched inverted index as an experimental feature in v23.1, we tested Apache Doris with the same dataset and SQL as described in the ClickHouse [blog](https://clickhouse.com/blog/clickhouse-search-with-inverted-indices), and compared performance of the two under the same testing resource, case, and tool. + +**Data**: 6.7G, 28.73 million rows, the Hacker News dataset, Parquet format + +**Query**: 3 keyword searches, counting the number of occurrence of the keywords "ClickHouse", "OLAP" OR "OLTP", and "avx" AND "sve". + +**Environment**: 1 × 16C 64G cloud virtual machine + +**Result**: Apache Doris was **4.7 times, 12 times, 18.5 times** faster than ClickHouse in the three queries, respectively. + + + +## Usage & Example + +**Dataset**: one million comment records from Hacker News + +- **Step 1**: Specify inverted index to the data table upon table creation. + +- **Parameters**: + +- - INDEX idx_comment (`comment`): create an index named "idx_comment" comment for the "comment" column + - USING INVERTED: specify inverted index for the table + - PROPERTIES("parser" = "english"): specify the tokenization language to English + +```SQL +CREATE TABLE hackernews_1m +( + `id` BIGINT, + `deleted` TINYINT, + `type` String, + `author` String, + `timestamp` DateTimeV2, + `comment` String, + `dead` TINYINT, + `parent` BIGINT, + `poll` BIGINT, + `children` Array<BIGINT>, + `url` String, + `score` INT, + `title` String, + `parts` Array<INT>, + `descendants` INT, + INDEX idx_comment (`comment`) USING INVERTED PROPERTIES("parser" = "english") COMMENT 'inverted index for comment' +) +DUPLICATE KEY(`id`) +DISTRIBUTED BY HASH(`id`) BUCKETS 10 +PROPERTIES ("replication_num" = "1"); +``` + +Note: You can add index to an existing table via `ADD INDEX idx_comment ON hackernews_1m(`comment`) USING INVERTED PROPERTIES("parser" = "english") `. Different from that of smart index and secondary index, the creation of inverted index only involves the reading of the comment column, so it can be much faster. + +**Step 2**: Retrieve the words"OLAP" and "OLTP" in the comment column with `MATCH_ALL`. The response time here was 1/10 of that in hard matching with `like`. (The performance gap widens as data volume increases.) + +```SQL +mysql> SELECT count() FROM hackernews_1m WHERE comment LIKE '%OLAP%' AND comment LIKE '%OLTP%'; ++---------+ +| count() | ++---------+ +| 15 | ++---------+ +1 row in set (0.13 sec) + +mysql> SELECT count() FROM hackernews_1m WHERE comment MATCH_ALL 'OLAP OLTP'; ++---------+ +| count() | ++---------+ +| 15 | ++---------+ +1 row in set (0.01 sec) +``` + +For more feature introduction and usage guide, see documentation: [Inverted Index](https://doris.apache.org/docs/dev/data-table/index/inverted-index/) + +## Wrap-up + +In a word, what contributes to Apache Doris' 10-time higher cost-effectiveness than Elasticsearch is its OLAP-tailored optimizations for inverted indexing, supported by the columnar storage engine, massively parallel processing framework, vectorized query engine, and cost-based optimizer of Apache Doris. + +As proud as we are about our own inverted indexing solution, we understand that self-published benchmarks can be controversial, so we are open to [feedback](https://t.co/KcxAtAJZjZ) from any third-party users and see how [Apache Doris](https://github.com/apache/doris) works in real-world cases. \ No newline at end of file diff --git a/i18n/zh-CN/docusaurus-plugin-content-blog/Inverted Index.md b/i18n/zh-CN/docusaurus-plugin-content-blog/Inverted Index.md new file mode 100644 index 00000000000..3df7abb207c --- /dev/null +++ b/i18n/zh-CN/docusaurus-plugin-content-blog/Inverted Index.md @@ -0,0 +1,281 @@ +--- +{ + 'title': '从 Elasticsearch 到 Apache Doris,10 倍性价比的新一代日志存储分析平台', + 'summary': "Apache Doris 借鉴了信息检索的核心技术,在存储引擎上实现了面向 AP 场景优化的高性能倒排索引,对于字符串类型的全文检索和普通数值、日期等类型的等值、范围检索具有更高效的支持,相较于 Elasticsearch 实现性价比 10 余倍的提升,以此为日志存储与分析场景提供了更优的选择。", + 'date': '2023-05-26', + 'author': '肖康', + 'tags': ['技术解析'], +} + + +--- + +<!-- +Licensed to the Apache Software Foundation (ASF) under one +or more contributor license agreements. See the NOTICE file +distributed with this work for additional information +regarding copyright ownership. The ASF licenses this file +to you under the Apache License, Version 2.0 (the +"License"); you may not use this file except in compliance +with the License. You may obtain a copy of the License at + + http://www.apache.org/licenses/LICENSE-2.0 + +Unless required by applicable law or agreed to in writing, +software distributed under the License is distributed on an +"AS IS" BASIS, WITHOUT WARRANTIES OR CONDITIONS OF ANY +KIND, either express or implied. See the License for the +specific language governing permissions and limitations +under the License. +--> + + + + + +**作者介绍**:肖康,[SelectDB](https://cn.selectdb.com/) 技术副总裁 + +# 导语 + +日志数据的处理与分析是最典型的大数据分析场景之一,过去业内以 Elasticsearch 和 Grafana Loki 为代表的两类架构难以同时兼顾高吞吐实时写入、低成本海量存储、实时文本检索的需求。Apache Doris 借鉴了信息检索的核心技术,在存储引擎上实现了面向 AP 场景优化的高性能倒排索引,对于字符串类型的全文检索和普通数值、日期等类型的等值、范围检索具有更高效的支持,相较于 Elasticsearch 实现性价比 10 余倍的提升,以此为日志存储与分析场景提供了更优的选择。 + + +# 日志数据分析的需求与特点 + +日志数据在企业大数据中非常普遍,其体量往往在企业大数据体系中占据非常高的比重,包括服务器、数据库、网络设备、IoT 物联网设备产生的系统运维日志,与此同时还包含了用户行为埋点等业务日志。 + +日志数据对于保障系统稳定运行和业务发展至关重要:基于日志的监控告警可以发现系统运行风险,及时预警;在故障排查过程中,实时日志检索能帮助工程师快速定位到问题,尽快恢复服务;日志报表能通过长历史统计发现潜在趋势。而用户埋点日志数据则是用户行为分析以及智能推荐业务所依赖的决策基础,有助于用户需求洞察与体验优化以及后续的业务流程改进。 + +由于其在业务中能发挥的重要意义,因此构建统一的日志分析平台,提供对日志数据的存储、高效检索以及快速分析能力,成为企业挖掘日志数据价值的关键一环。而日志数据和应用场景往往呈现如下的特点: + +- 数据增长快:每一次用户操作、系统事件都会触发新的日志产生,很多企业每天新增日志达到几十甚至几百亿条,对日志平台的写入吞吐要求很高; +- 数据总量大:由于自身业务和监管等需要,日志数据经常要存储较长的周期,因此累积的数据量经常达到几百 TB 甚至 PB 级,而较老的历史数据访问频率又比较低,面临沉重的存储成本压力; +- 时效性要求高:在故障排查等场景需要能快速查询到最新的日志,分钟级的数据延迟往往无法满足业务极高的时效性要求,因此需要实现日志数据的实时写入与实时查询。 + +这些日志数据和应用场景的特点,为承载存储和分析需求的日志平台提出了如下挑战: + +- 高吞吐实时写入:既需要保证日志流量的大规模写入,又要支持低延迟可见; +- 低成本大规模存储:既要存储大量的数据,又要降低存储成本; +- 支持文本检索的实时查询:既要能支持日志文本的全文检索,又要做到实时查询响应; + + +# 业界日志存储分析解决方案 + +当前业界有两种比较典型的日志存储与分析架构,分别是以 Elasticsearch 为代表的倒排索引检索架构以及以 Loki 为代表的轻量索引/无索引架构,如果我们从实时写入吞吐、存储成本、实时交互式查询性能等几方面进行对比,不难发现以下结论: + +- 以 ES 为代表的倒排索引检索架构,支持全文检索、查询性能好,因此在日志场景中被业内大规模应用,但其仍存在一些不足,包括实时写入吞吐低、消耗大量资源构建索引,且需要消耗巨大存储成本; +- 以 Loki 为代表的轻量索引或无索引架构,实时写入吞吐高、存储成本较低,但是检索性能慢、关键时候查询响应跟不上,性能成为制约业务分析的最大掣肘。 + +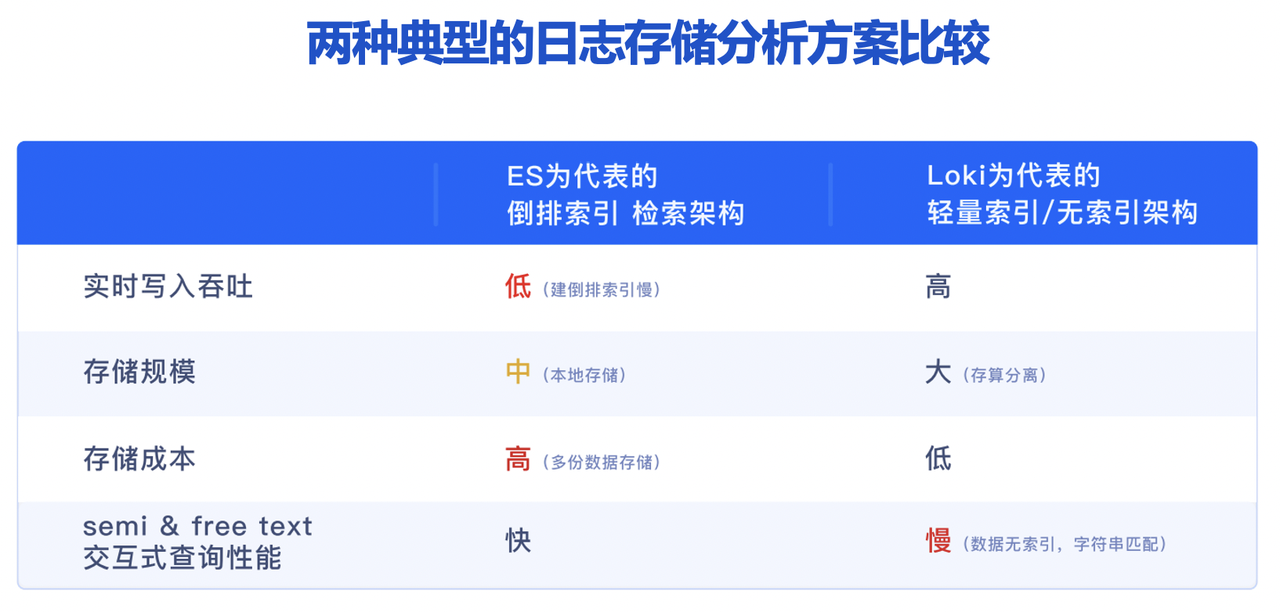 + +ES 在日志场景的优势在于全文检索能力,能快速从海量日志中检索出匹配关键字的日志,其底层核心技术是倒排索引(Inverted Index)。 + +倒排索引是一种用于快速查找文档中包含特定单词或短语的数据结构,最早应用于信息检索领域。如下图所示,在数据写入时,倒排索引可以将每一行文本进行分词,变成一个个词(Term),然后构建词(Term) -> 行号列表(Posting List) 的映射关系,将映射关系按照词进行排序存储。当需要查询某个词在哪些行出现的时候,先在 词 -> 行号列表 的有序映射关系中查找词对应的行号列表,然后用行号列表中的行号去取出对应行的内容。这样的查询方式,可以避免遍历对每一行数据进行扫描和匹配,只需要访问包含查找词的行,在海量数据下性能有数量级的提升。 + +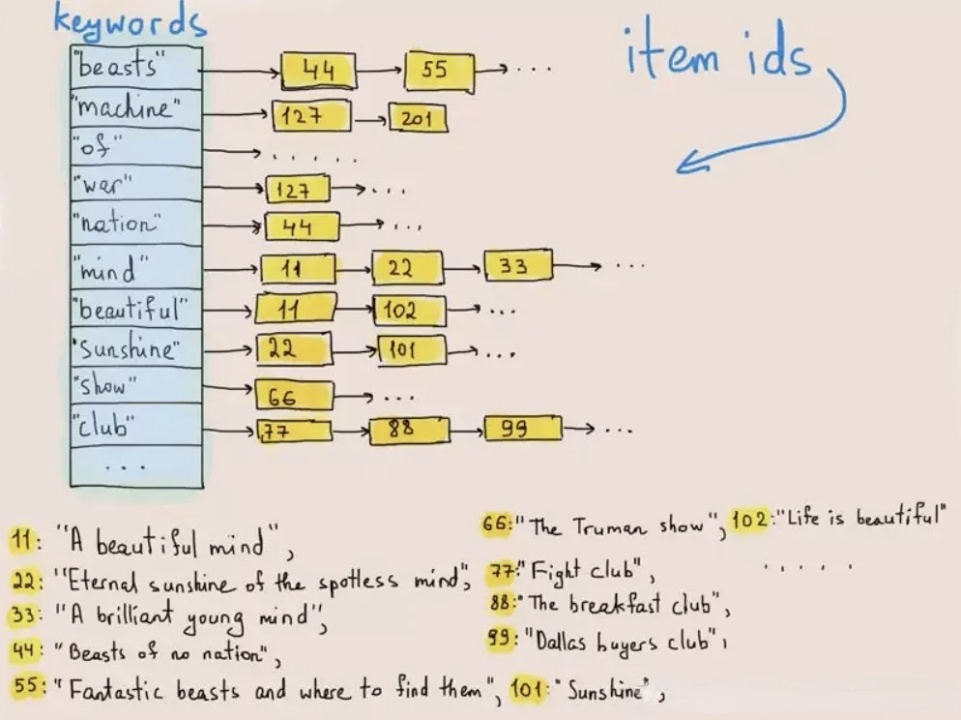 + +图:倒排索引原理示意 + + + +倒排索引为 ES 带来快速检索能力的同时,也付出了写入速度吞吐低和存储空间占用高的代价——由于数据写入时倒排索引需要进行分词、词典排序、构建倒排表等 CPU 和内存密集型操作,导致写入吞吐大幅下降。而从存储成本角度考虑,ES 会存储原始数据和倒排索引,为了加速分析可能还需要额外存储一份列存数据,因此 3 份冗余也会导致更高的存储空间占用。 + +Loki 则放弃了倒排索引,虽然带来来写入吞吐和存储空间的优势,但是损失了日志检索的用户体验,在关键时刻不能发挥快速查日志的作用。成本虽然有所降低,但是没有真正解决用户的问题。 + +# 更高性价比的日志存储分析解决方案 + +从以上方案对比可知,以 Elasticsearch 为代表的倒排索引检索架构以及以 Loki 为代表的轻量索引/无索引架构无法同时兼顾 高吞吐、低存储成本和实时高性能的要求,只能在某一方面或某几方面做权衡取舍。如果在保持倒排索引的文本检索性能优势的同时,大幅提升系统的写入速度与吞吐量并降低存储资源成本,是否日志场景所面临的困境就迎刃而解呢?答案是肯定的。 + +如果我们希望使用 Apache Doris 来更好解决日志存储与分析场景的痛点,其实现路径也非常清晰——**在数据库内部增加倒排索引、以满足字符串类型的全文检索和普通数值/日期等类型的等值、范围检索,同时进一步优化倒排索引的查询性能、使其更加契合日志数据分析的场景需求**。 + +在同样实现倒排索引的情况下,相较于 ES, Apache Doris 怎么做到更高的性能表现呢?或者说现有倒排索引的优化空间有哪些呢? + +- ES 基于 Apache Lucene 构建倒排索引,Apache Lucene 自 2000 年开源至今已有超过 20 年的历史,设计之初主要面向信息检索领域、功能丰富且复杂,而日志和大多数 OLAP 场景只需要其核心功能,包括分词、倒排表等,而相关度排序等并非强需求,因此存在进一步功能简化和性能提升的空间; +- ES 和 Apache Lucene 均采用 Java 实现,而 Apache Doris 存储引擎和执行引擎采用 C++ 开发并且实现了全面向量化,相对于 Java 实现具有更好的性能; +- 倒排索引并不能决定性能表现的全部,作为一个高性能、实时的 OLAP 数据库,Apache Doris 的列式存储引擎、MPP 分布式查询框架、向量化执行引擎以及智能 CBO 查询优化器,相较于 ES 更为高效。 + +通过在 [Apache Doris 2.0.0 最新版本](https://github.com/apache/doris/releases/tag/2.0.0-alpha1)的探索与持续优化,在相同硬件配置和数据集的测试表现上,Apache Doris 在数据库内核实现高性能倒排索引后,相对于 ES 实现了日志数据写入速度提升 4 倍、存储空间降低 80%、查询性能提升 2 倍,再结合 Apache Doris 2.0.0 版本引入的冷热数据分离特性,整体性价比提升 10 倍以上! + +接下来我们进一步介绍设计与实现细节。 + +# 高性能倒排索引的设计与实现 + +业界各类系统为了支持全文检索和任意列索引,往往有两种实现方式:一是通过外接索引系统来实现,原始数据存储在原系统中、索引存储在独立的索引系统中,两个系统通过数据的 ID 进行关联。数据写入时会同步写入到原系统和索引系统,索引系统构建索引后不存储完整数据只保留索引。查询时先从索引系统查出满足过滤条件的数据 ID 集合,然后用 ID 集合去原系统查原始数据。 + +这种架构的优势是实现简单,借力外部索引系统,对原有系统改动小。但是问题也很明显: + +- 数据写入两个系统,异常有数据不一致的问题,也存在一定冗余存储; +- 查询需在两个系统进行网络交互有额外开销,数据量大时用 ID 集合去原系统查性能比较低; +- 维护两套系统的复杂度高,将系统的复杂性从开发测转移到运维测; + +而另一种方式则是直接在系统中内置倒排索引,尽管技术难度更高,但性能更好、且无需花费额外的系统维护成本,对用户更加友好,这也是 Apache Doris 所选择的方式。 + +### 数据库内置倒排索引 + +在选择了在数据库内核中内置倒排索引后,我们需要进一步对 Apache Doris 索引结构进行分析,判断能否通过在已有索引基础上进行拓展来实现。 + +Apache Doris 现有的索引存储在 Segment 文件的 Index Region 中,按照适用场景可以分为跳数索引和点查索引两类: + +- 跳数索引:包括 ZoneMap 索引和 Bloom Filter 索引。 + + - ZoneMap 索引对每一个数据块和文件保存 Min/Max/isnull 等汇总信息,可以用于等值、范围查询的粗粒度过滤,只能排除不满足查询条件的数据块和文件,不能定位到行,也不支持文本分词。 + - BloomFilter 索引也是数据块和文件级别的索引,通过 Bloom Filter 判断某个值是否在数据块和文件中,同样不能定位到行、不支持文本分词; + +- 点查索引:包括 ShortKey 前缀排序索引和 Bitmap 索引。 + + - ShortKey 在排序的基础上,根据给定的前缀列实现快速查询数据的索引方式,能够对前缀索引的列进行等值、范围查询,但不支持文本分词,另外由于数据要按前缀索引排序、因此一个表只允许一组前缀索引。 + - Bitmap 索引记录数据值 -> 行号 Bitmap 的有序映射,是一种很基础的倒排索引,但是索引结构比较简单、查询效率不高、不支持文本分词。 + +原有索引结构很难满足日志场景实时文本检索的需求,因此设计了全新的倒排索引。倒排索引在设计和实现上我们采取了无侵入的方式、不改变 Segment 数据文件格式,而是增加了新的 Inverted Index File,逻辑上在 Table 的 Column 级别。具体流程如下: + +- 数据写入和 Compaction 阶段:在写 Segment 文件的同时,同步写入一个 Inverted Index 文件,文件路径由 Segment ID + Index ID 决定。写入 Segment 的 Row 和 Index 中的 Doc 一一对应,由于同步顺序写入,Segment 中的 Rowid 和 Index 中的 Docid 完全对应。 +- 查询阶段:如果查询 Where 条件中有建了倒排索引的列,会自动去 Index 文件中查询,返回满足条件的 Docid List,将 Docid List 一一对应的转成 Rowid Bitmap,然后走 Doris 通用的 Rowid 过滤机制只读取满足条件的行,达到查询加速的效果。 + +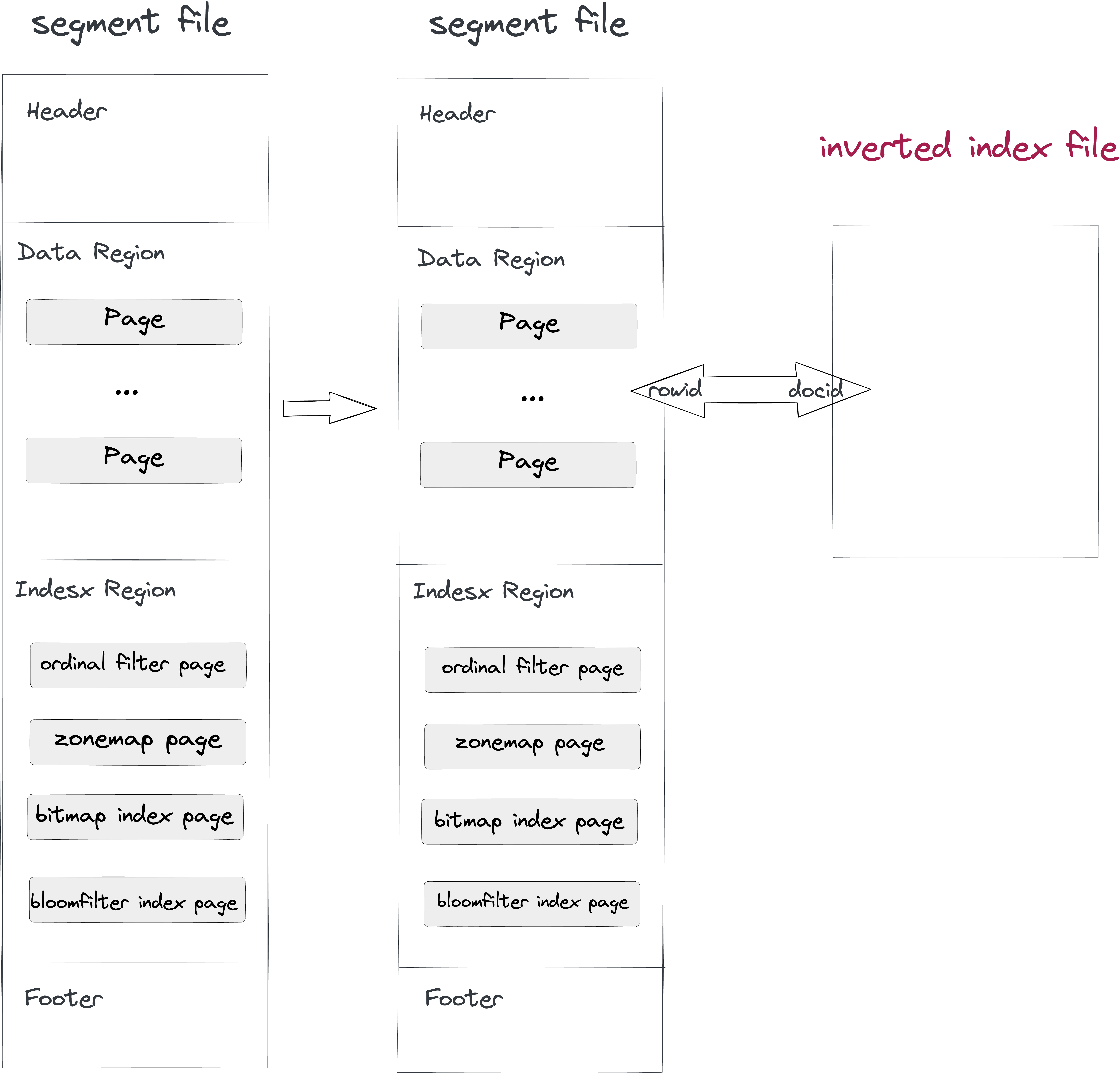 + +图:Doris倒排索引架构图 + + +这个设计的好处是已有的数据文件无需修改,可以做到兼容升级,而且增减索引不影响数据文件和其他索引,用户增建索引没有负担。 + +### 通用倒排索引优化 + +**C++和向量化实现** + +Apache Doris 使用 [CLucene](https://clucene.sourceforge.net/) 作为底层的倒排索引库,CLucene 是一个用 C++ 实现的高性能、稳定的 Lucene 倒排索引库,它的功能比较完整,支持分词和自定义分词算法,支持全文检索查询和等值、范围查询。 + +Apache Doris 的存储模块和 CLucene 都用 C++ 实现,避免了Java Lucene 的 JVM GC 等开销,同样的计算 C++ 实现相对于 Java 性能优势明显,而且更利于做向量化加速。Doris 倒排索引进行了向量化优化,包括分词、倒排表构建、查询等,性能得到进一步提升。整体来看 Doris 的倒排索引写入速度可以超过单核 20MB/s,而 ES 的单核写入速度不到 5MB/s,有 4 倍的性能优势。 + +**列式存储和压缩** + +Lucene 本身是文档存储模型,主数据采用行存,而 Doris 中不同列的倒排索引是相互独立的,因此倒排索引文件也采用列式存储,有利于向量化构建索引和提高压缩率。 + +采用压缩比高且速度快的 ZSTD,通常可以达到 5 ~10倍的压缩比,与常用的GZIP压缩相比有50%以上的空间节省且速度更快。 + +**BKD 索引与** **数值、日期类型** **列优化** + +针对数值、日期类型的列,我们还实现了 BKD 索引,可以对范围查询提高性能,存储空间也相对于转成定长字符串更加高效,具有以下主要特性和优势: + +1. 高效范围查询:BKD 索引采用多维数据结构,为范围查询带来高效率。它能迅速定位数值或日期类型列中所需的数据范围,降低查询时间复杂度。 +1. 存储空间优化:与其他索引方法相比,BKD 索引在存储空间使用上更高效。通过聚合并压缩相邻数据块,减少索引所需存储空间,降低存储成本。 +1. 多维数据支持:BKD 索引具备良好扩展性,支持多维数据类型,如地理坐标(GEO point)和范围(Range),使其在处理复杂数据类型时具有高适应性。 + +此外,我们在原有 BKD 索引能力基础上进行了进一步拓展: + +1. 优化低基数场景:针对数值分布集中、单个数值倒排列表较多的低基数场景,我们调整了针对性的压缩算法,降低大量倒排表解压缩和反序列化所带来的CPU性能消耗。 +1. 预查询技术:针对查询结果命中数较高的场景,我们采用预查询技术进行命中数预估。若命中数显著超过阈值,可跳过索引查询,直接利用Doris在大数据量查询下的技术优势进行数据过滤。 + + +### 面向 OLAP 的倒排索引优化 + +日志存储和分析场景对检索的需求很简单,不需要特别复杂的功能(比如相关性排序),更需要降低存储成本和快速按照条件查出数据。因此,在面对海量数据的写入和查询时,Apache Doris 还针对 OLAP 数据库的特点优化了倒排索引的结构,使其更加简洁高效。例如: + +- 在写入流程保证不会多个线程写入一个索引,从而避免写入时多线程锁竞争的开销; +- 在存储结构上去掉了不必要的正排、norm 等文件,减少写入 IO 开销和存储空间占用; +- 查询过程中简化相关性打分和排序逻辑,降低不必要的开销,提升查询性能。 + + +针对日志等数据有按时间分区、历史数据访问频度低的特点,基于独立的索引文件设计,Apache Doris 还将在后续的版本中提供更细粒度、更灵活的索引管理功能: + +- 指定分区构建倒排索引,比如新增一个索引的时候指定最近7天的日志构建索引,历史数据不建索引 +- 指定分区删除倒排索引,比如删除超过1个月的日志的索引,释放访问频度低的索引存储空间 + + +# 性能测试 + +高性能是 Apache Doris 倒排索引设计和实现的首要出发点,我们通过公开的测试数据集分别与 ES 以及 Clickhouse 进行性能测试,测试效果如下: + +### vs Elasticsearch + +我们采用了 ES 官方的性能测试 Benchmark esrally 并使用其中的 HTTP Logs 日志,在同样的硬件资源、数据、测试Case 以及测试工具下,记录并对比各自的数据写入时间、吞吐以及查询延迟。 + +- 测试数据:esrally HTTP Logs track 中自带测试数据集,1998 年 World Cup HTTP Server Logs,未压缩前 32G、共 2.47 亿行、单行平均长度 134 字节; +- 测试查询:esrally HTTP Logs 测试关键词检索、范围查询、聚合、排序等 11 个 Query,所有查询跑 100 次串行执行; +- 测试环境:3 台 16C 64G 云主机组成的集群。 + +在最终的测试结果中,**Doris 写入速度是 ES 的 4.2 倍**、达到 550 MB/s,写入后的数据压缩比接近 1:10、**存储空间** **节省** **超** ******80%** **,查询耗时下降 57%、查询性能是 ES 的 2.3 倍。加上冷热数据分离降低冷数据存储成本,整体相较 ES 实现 10倍以上的性价比提升。** + +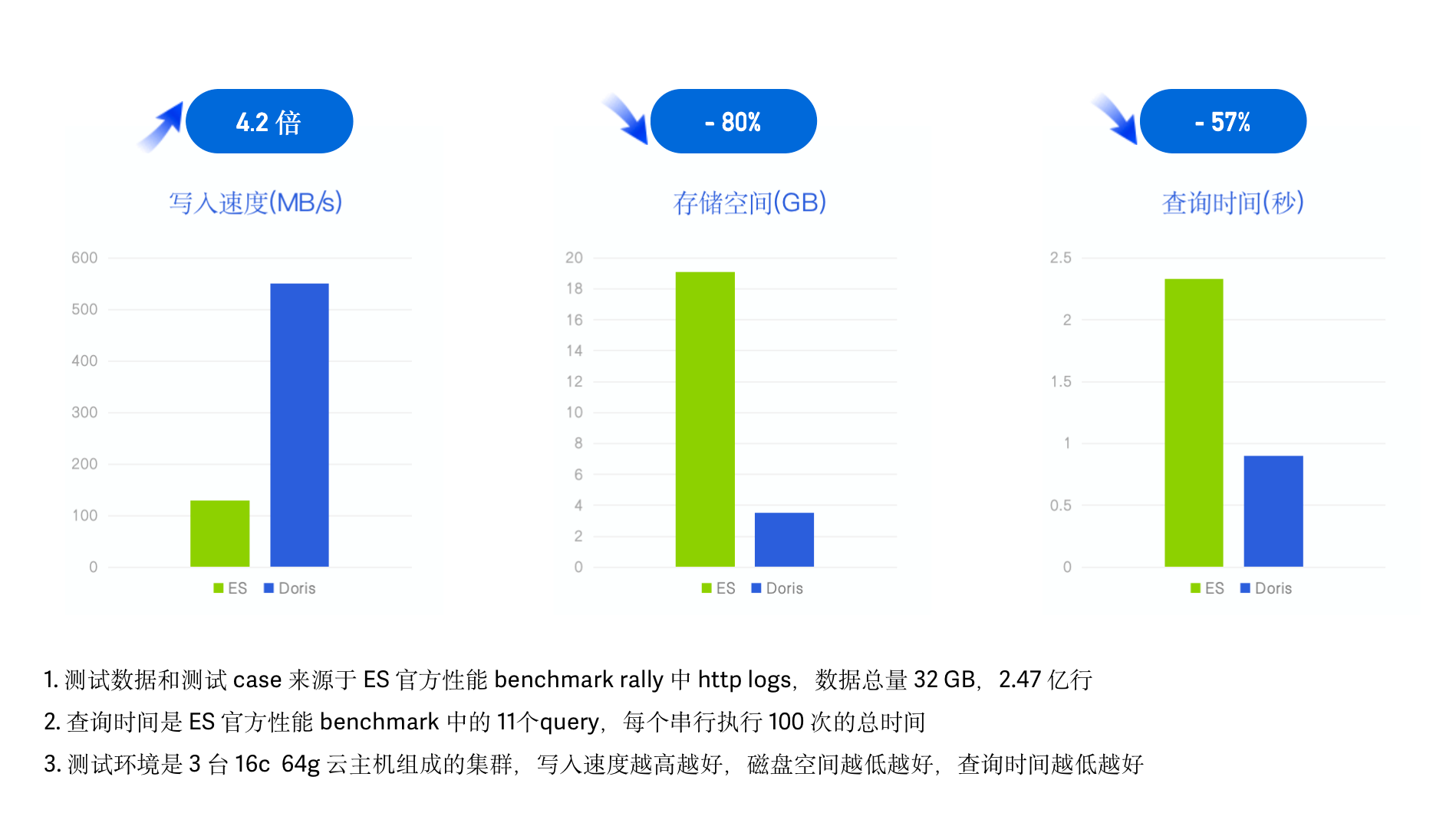 + +### vs Clickhouse + +Clickhouse 近期的 v23.1 版本也引入了类似 Feature,将倒排索引作为实验性功能发布,因此我们同样进行了跟 Clickhouse 倒排索引的性能对比。在本次测试中,我们采用了 Clickhouse 官方 Inverted Index 介绍博客中使用的 Hacker News 样例数据以及查询 SQL ,同样保持相同的物理资源、数据、测试 Case 以及测试工具。 + +(参考文章:https://clickhouse.com/blog/clickhouse-search-with-inverted-indices) + +- 测试数据:Hacker News 2873 万条数据,6.7G,Parquet 格式; +- 测试查询:3 个查询,分别查询 'clickhouse'、'olap' OR 'oltp'、'avx' AND 'sve' 等关键字出现的次数; +- 测试机器:1 台 16C 64G 云主机 + +在最终的测试结果中,3 个 SQL **Apache Doris 的查询性能分别是 Clickhouse 的 4.7 倍、12.0 倍以及 18.5 倍**,有明显的性能优势。 + +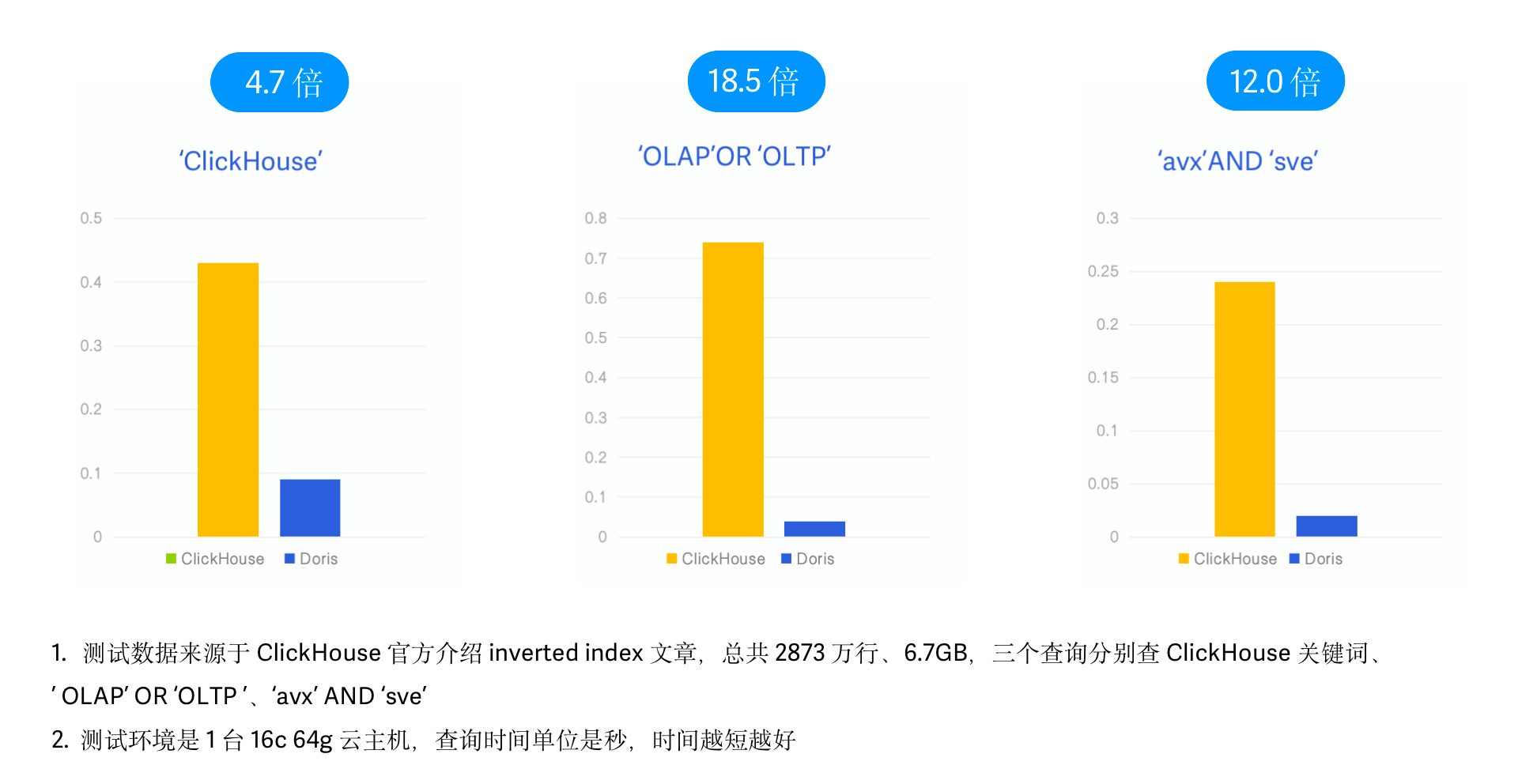 + +# 如何使用 + +下面以一个 Hacker News 100 万条测试数据的示例展示 Doris 如何利用倒排索引实现高效的日志分析。 + +1. 建表时指定索引 + + 1. INDEX idx_comment (`comment`) 指定对 comment 列建一个名为 idx_comment 的索引 + 1. USING INVERTED 指定索引类型为倒排索引 + 1. PROPERTIES("parser" = "english") 指定分词类型为英文分词 + +``` +CREATE TABLE hackernews_1m +( + `id` BIGINT, + `deleted` TINYINT, + `type` String, + `author` String, + `timestamp` DateTimeV2, + `comment` String, + `dead` TINYINT, + `parent` BIGINT, + `poll` BIGINT, + `children` Array<BIGINT>, + `url` String, + `score` INT, + `title` String, + `parts` Array<INT>, + `descendants` INT, + INDEX idx_comment (`comment`) USING INVERTED PROPERTIES("parser" = "english") COMMENT 'inverted index for comment' +) +DUPLICATE KEY(`id`) +DISTRIBUTED BY HASH(`id`) BUCKETS 10 +PROPERTIES ("replication_num" = "1"); +``` + +注:对于已经存在的表,也可以通过 `` ADD INDEX idx_comment ON hackernews_1m(`comment`) USING INVERTED PROPERTIES("parser" = "english") ``来增加索引。值得一提的是,和 Doris 原先存储在 Segment 数据文件中的智能索引和二级索引相比,增加倒排索引的过程只会读 comment 列构建新的倒排索引文件,不会读写原有的其他数据,效率有明显提升。 + +2. 导入数据后查询,使用 `MATCH_ALL` 在 comment 这一列上匹配 OLAP 和 OLTP 两个词,和 LIKE 扫描硬匹配相比,查询性能有十余倍的提升。(这仅是 100 万条数据下的测试效果,而随着数据量增大、性能提升越明显) + +``` +mysql> SELECT count() FROM hackernews_1m WHERE comment LIKE '%OLAP%' AND comment LIKE '%OLTP%'; ++---------+ +| count() | ++---------+ +| 15 | ++---------+ +1 row in set (0.13 sec) + +mysql> SELECT count() FROM hackernews_1m WHERE comment MATCH_ALL 'OLAP OLTP'; ++---------+ +| count() | ++---------+ +| 15 | ++---------+ +1 row in set (0.01 sec) +``` + +更多详细功能介绍和测试步骤可以参考Apache Doris [倒排索引官方文档](https://doris.apache.org/zh-CN/docs/dev/data-table/index/inverted-index) 。 + +# 总结 + +通过内置高性能倒排索引,Apache Doris 对于字符串类型的全文检索和普通数值、日期等类型的等值、范围检索具有更高效的支持,进一步提升了数据查询的效率和准确性,对于大规模日志数据查询分析有了更好的性能表现,为需要检索能力的用户提供了更高性价比的选择。 + +目前倒排索引已经支持了 String、Int、Decimal、Datetime 等常用 Scalar 数据类型和 Array 数组类型,后续还会增加对 JSONB、Map 等复杂数据类型的支持。而 BKD 索引可以支持多维度类型的索引,为未来 Doris 增加 GEO 地理位置数据类型和索引打下了基础。与此同时 Apache Doris 在半结构化数据分析方面还有更多能力扩展,比如自动根据导入数据扩展表结构的 Dynamic Table、丰富的复杂数据类型(Array、Map、Struct、JSONB)以及高性能字符串匹配算法等。 + +除倒排索引以外,[Apache Doris 在 2.0.0 Alpha 版本](https://github.com/apache/doris/releases/tag/2.0.0-alpha1)中还实现了单节点数万 QPS 的高并发点查询能力、基于对象存储的冷热数据分离、基于代价模型的全新查询优化器以及 Pipeline 执行引擎等,欢迎大家下载体验。高并发点查询的详细介绍可以查看 SelectDB 技术团队过往发布的技术博客,其他功能的使用介绍请参考社区官方文档,同时也敬请持续关注我们后续发布的特性解读系列文章。 + +为了让用户可以体验社区开发的最新特性,同时保证最新功能可以收获到更广范围的使用反馈,我们建立了 2.0.0 版本的专项支持群,欢迎广大社区用户在使用最新版本过程中多多反馈使用意见,帮助 Apache Doris 持续改进,[通过此处填写申请加入专项支持群。](https://wenjuan.feishu.cn/m/cfm?t=sF2FZOL1KXKi-m73g) diff --git a/static/images/Inverted_1.png b/static/images/Inverted_1.png new file mode 100644 index 00000000000..456c7be3df2 Binary files /dev/null and b/static/images/Inverted_1.png differ diff --git a/static/images/Inverted_2.png b/static/images/Inverted_2.png new file mode 100644 index 00000000000..5fd028f2d04 Binary files /dev/null and b/static/images/Inverted_2.png differ diff --git a/static/images/Inverted_3.png b/static/images/Inverted_3.png new file mode 100644 index 00000000000..927b25de16c Binary files /dev/null and b/static/images/Inverted_3.png differ diff --git a/static/images/Inverted_4.png b/static/images/Inverted_4.png new file mode 100644 index 00000000000..701d177b3f5 Binary files /dev/null and b/static/images/Inverted_4.png differ diff --git a/static/images/Inverted_5.png b/static/images/Inverted_5.png new file mode 100644 index 00000000000..0a4dd0c0861 Binary files /dev/null and b/static/images/Inverted_5.png differ diff --git a/static/images/Inverted_6.png b/static/images/Inverted_6.png new file mode 100644 index 00000000000..fc2722d027d Binary files /dev/null and b/static/images/Inverted_6.png differ --------------------------------------------------------------------- To unsubscribe, e-mail: commits-unsubscr...@doris.apache.org For additional commands, e-mail: commits-h...@doris.apache.org