This is an automated email from the ASF dual-hosted git repository. luzhijing pushed a commit to branch master in repository https://gitbox.apache.org/repos/asf/doris-website.git
The following commit(s) were added to refs/heads/master by this push: new d67a11c1ec5 Add Douyu blog (#219) d67a11c1ec5 is described below commit d67a11c1ec525c10728aaa5bc635324ff5cfc91b Author: Hu Yanjun <100749531+httpshir...@users.noreply.github.com> AuthorDate: Sat May 6 22:09:36 2023 +0800 Add Douyu blog (#219) --- blog/Douyu.md | 97 +++++++++++ blog/High_concurrency.md | 2 +- i18n/zh-CN/docusaurus-plugin-content-blog/Douyu.md | 192 +++++++++++++++++++++ static/images/Douyu_1.png | Bin 0 -> 139532 bytes static/images/Douyu_2.png | Bin 0 -> 207516 bytes static/images/Douyu_3.png | Bin 0 -> 211500 bytes static/images/Douyu_4.png | Bin 0 -> 166334 bytes static/images/Douyu_5.png | Bin 0 -> 156514 bytes static/images/Douyu_6.png | Bin 0 -> 160932 bytes 9 files changed, 290 insertions(+), 1 deletion(-) diff --git a/blog/Douyu.md b/blog/Douyu.md new file mode 100644 index 00000000000..52ca06552f4 --- /dev/null +++ b/blog/Douyu.md @@ -0,0 +1,97 @@ +--- +{ + 'title': 'Zipping up the Lambda Architecture for 40% Faster Performance', + 'summary': "Instead of pooling real-time and offline data after they are fully ready for queries, Douyu engineers use Apache Doris to share part of the pre-query computation burden.", + 'date': '2023-05-05', + 'author': 'Tongyang Han', + 'tags': ['Best Practice'], +} + +--- + +<!-- +Licensed to the Apache Software Foundation (ASF) under one +or more contributor license agreements. See the NOTICE file +distributed with this work for additional information +regarding copyright ownership. The ASF licenses this file +to you under the Apache License, Version 2.0 (the +"License"); you may not use this file except in compliance +with the License. You may obtain a copy of the License at + + http://www.apache.org/licenses/LICENSE-2.0 + +Unless required by applicable law or agreed to in writing, +software distributed under the License is distributed on an +"AS IS" BASIS, WITHOUT WARRANTIES OR CONDITIONS OF ANY +KIND, either express or implied. See the License for the +specific language governing permissions and limitations +under the License. +--> + +Author: Tongyang Han, Senior Data Engineer at Douyu + +The Lambda architecture has been common practice in big data processing. The concept is to separate stream (real time data) and batch (offline data) processing, and that's exactly what we did. These two types of data of ours were processed in two isolated tubes before they were pooled together and ready for searches and queries. + + + +Then we run into a few problems: + +1. **Isolation of real-time and offline data warehouses** + 1. I know this is kind of the essence of Lambda architecture, but that means we could not reuse real-time data since it was not layered as offline data, so further customized development was required. +2. **Complex Pipeline from Data Sources to Data Application** + 1. Data had to go through multi-step processing before it reached our data users. As our architecture involved too many components, navigating and maintaining these tech stacks was a lot of work. +3. **Lack of management of real-time data sources** + 1. In extreme cases, this worked like a data silo and we had no way to find out whether the ingested data was duplicated or reusable. + +So we decided to "zip up" the Lambda architecture a little bit. By "zipping up", I mean to introduce an OLAP engine that is capable of processing, storing, and analyzing data, so real-time data and offline data converge a little earlier than they used to. It is not a revolution of Lambda, but a minor change in the choice of components, which made our real-time data processing 40% faster. + +## **Zipping up Lambda Architecture** + +I am going to elaborate on how this is done using our data tagging process as an example. + +Previously, our offline tags were produced by the data warehouse, put into a flat table, and then written in **HBase**, while real-time tags were produced by **Flink**, and put into **HBase** directly. Then **Spark** would work as the computing engine. + + + +The problem with this stemmed from the low computation efficiency of **Flink** and **Spark**. + +- **Real-time tag production**: When computing real-time tags that involve data within a long time range, Flink did not deliver stable performance and consumed more resources than expected. And when a task failed, it would take a really long time for checkpoint recovery. +- **Tag query**: As a tag query engine, Spark could be slow. + +As a solution, we replaced **HBase** and **Spark** with **Apache Doris**, a real-time analytic database, and moved part of the computational logic of the foregoing wide-time-range real-time tags from **Flink** to **Apache Doris**. + + + +Instead of putting our flat tables in HBase, we place them in Apache Doris. These tables are divided into partitions based on time sensitivity. Offline tags will be updated daily while real-time tags will be updated in real time. We organize these tables in the Aggregate Model of Apache Doris, which allows partial update of data. + +Instead of using Spark for queries, we parse the query rules into SQL for execution in Apache Doris. For pattern matching, we use Redis to cache the hot data from Apache Doris, so the system can respond to such queries much faster. + + + +## **Computational Pipeline of Wide-Time-Range Real-Time Tags** + +In some cases, the computation of wide-time-range real-time tags entails the aggregation of historical (offline) data with real-time data. The following figure shows our old computational pipeline for these tags. + + + +As you can see, it required multiple tasks to finish computing one real-time tag. Also, in complicated aggregations that involve a collection of aggregation operations, any improper resource allocation could lead to back pressure or waste of resources. This adds to the difficulty of task scheduling. The maintenance and stability guarantee of such a long pipeline could be an issue, too. + +To improve on that, we decided to move such aggregation workload to Apache Doris. + + + +We have around 400 million customer tags in our system, and each customer is attached with over 300 tags. We divide customers into more than 10,000 groups, and we have to update 5000 of them on a daily basis. The above improvement has sped up the computation of our wide-time-range real-time queries by **40%**. + +## Overwrite + +To atomically replace data tables and partitions in Apache Doris, we customized the [Doris-Spark-Connector](https://github.com/apache/doris-spark-connector), and added an "Overwrite" mode to the Connector. + +When a Spark job is submitted, Apache Doris will call an interface to fetch information of the data tables and partitions. + +- If it is a non-partitioned table, we create a temporary table for the target table, ingest data into it, and then perform atomic replacement. If the data ingestion fails, we clear the temporary table; +- If it is a dynamic partitioned table, we create a temporary partition for the target partition, ingest data into it, and then perform atomic replacement. If the data ingestion fails, we clear the temporary partition; +- If it is a non-dynamic partitioned table, we need to extend the Doris-Spark-Connector parameter configuration first. Then we create a temporary partition and take steps as above. + +## Conclusion + +One prominent advantage of Lambda architecture is the stability it provides. However, in our practice, the processing of real-time data and offline data sometimes intertwines. For example, the computation of certain real-time tags requires historical (offline) data. Such interaction becomes a root cause of instability. Thus, instead of pooling real-time and offline data after they are fully ready for queries, we use an OLAP engine to share part of the pre-query computation burden and mak [...] diff --git a/blog/High_concurrency.md b/blog/High_concurrency.md index 6a499918306..85d3240482c 100644 --- a/blog/High_concurrency.md +++ b/blog/High_concurrency.md @@ -4,7 +4,7 @@ 'summary': 'In the upcoming Apache Doris 2.0, we have optimized it for high-concurrency point queries. Long story short, it can achieve over 30,000 QPS for a single node.', 'date': '2023-04-14', 'author': 'Apache Doris', - 'tags': ['Tech Insights'], + 'tags': ['Tech Sharing'], } --- diff --git a/i18n/zh-CN/docusaurus-plugin-content-blog/Douyu.md b/i18n/zh-CN/docusaurus-plugin-content-blog/Douyu.md new file mode 100644 index 00000000000..e535d6e426d --- /dev/null +++ b/i18n/zh-CN/docusaurus-plugin-content-blog/Douyu.md @@ -0,0 +1,192 @@ +--- +{ + 'title': '赋能直播行业精细化运营,斗鱼基于 Apache Doris 的应用实践', + 'summary': "在目标驱动下,斗鱼在原有架构的基础上进行升级改造、引入 Apache Doris 构建了实时数仓体系,并在该基础上成功构建了标签平台以及多维分析平台", + 'date': '2023-05-05', + 'author': '韩同阳', + 'tags': ['最佳实践'], +} + +--- + +<!-- +Licensed to the Apache Software Foundation (ASF) under one +or more contributor license agreements. See the NOTICE file +distributed with this work for additional information +regarding copyright ownership. The ASF licenses this file +to you under the Apache License, Version 2.0 (the +"License"); you may not use this file except in compliance +with the License. You may obtain a copy of the License at + + http://www.apache.org/licenses/LICENSE-2.0 + +Unless required by applicable law or agreed to in writing, +software distributed under the License is distributed on an +"AS IS" BASIS, WITHOUT WARRANTIES OR CONDITIONS OF ANY +KIND, either express or implied. See the License for the +specific language governing permissions and limitations +under the License. +--> + +**导读:** 斗鱼是一家弹幕式直播分享网站,为用户提供视频直播和赛事直播服务。随着斗鱼直播、视频等业务的高速发展,用户增长和营收两大主营业务线对精细化运营的需求越发地迫切,各个细分业务场景对用户的差异化分析诉求也越发的强烈。为更好满足业务需求,斗鱼在 2022 年引入了 [Apache Doris](http://doris.apache.org) 构建了一套比较相对完整的实时数仓架构,并在该基础上成功构建了标签平台以及多维分析平台,在此期间积累了一些建设及实践经验通过本文分享给大家。 + + + + +作者**|**斗鱼资深大数据工程师、OLAP 平台负责人 韩同阳 + +斗鱼是一家弹幕式直播分享网站,为用户提供视频直播和赛事直播服务。斗鱼以游戏直播为主,也涵盖了娱乐、综艺、体育、户外等多种直播内容。随着斗鱼直播、视频等业务的高速发展,用户增长和营收两大主营业务线对精细化运营的需求越发地迫切,各个细分业务场景对用户的差异化分析诉求也越发的强烈,例如增长业务线需要在各个活动(赛事、专题、拉新、招募等)中针对不同人群进行差异化投放,营收业务线需要根据差异化投放的效果及时调整投放策略。 + + +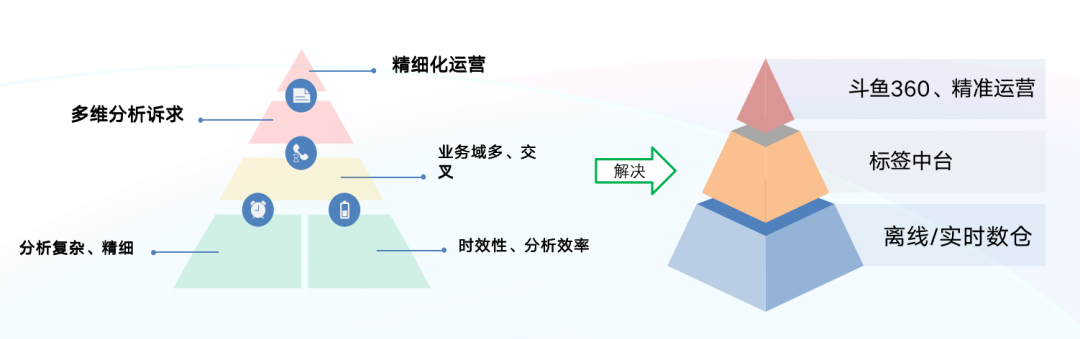 + +根据业务场景的诉求和精细化运营的要求,我们从金字塔自下而上来看,需求大致可以分为以下几点: + +- 分析需求更加复杂、精细化,不再满足简单的聚合分析;数据时效性要求更高,不满足于 T+1 的分析效率,期望实现近实时、实时的分析效率。 +- 业务场景多,细分业务场景既存在独立性、又存在交叉性,例如:针对某款游戏进行专题活动投放(主播、用户),进行人群圈选、AB 实验等,需要标签/用户画像平台支持。 +- 多维数据分析的诉求强烈,需要精细化运营的数据产品支持。 + +**为更好解决上述需求,我们的初步目标是:** + +- 构建离线/实时数仓,斗鱼的离线数仓体系已成熟,希望此基础上构建一套实时数仓体系; +- 基于离线/实时数仓构建通用的标签中台(用户画像平台),为业务场景提供人群圈选、AB实验等服务; +- 在标签平台的基础上构建适用于特定业务场景的多维分析和精细化运营的数据产品。 + +在目标驱动下,斗鱼在原有架构的基础上进行升级改造、**引入 [Apache Doris](https://github.com/apache/doris) 构建了实时数仓体系,并在该基础上成功构建了标签平台以及多维分析平台**,在此期间积累了一些建设及实践经验通过本文分享给大家。 + + + +# 原有实时数仓架构 + +斗鱼从 2018 年开始探索实时数仓的建设,并尝试在某些垂直业务领域应用,但受制于人力的配置及流计算组件发展的成熟度,直到 2020 年第一版实时数据架构才构建完成,架构图如下图所示: + +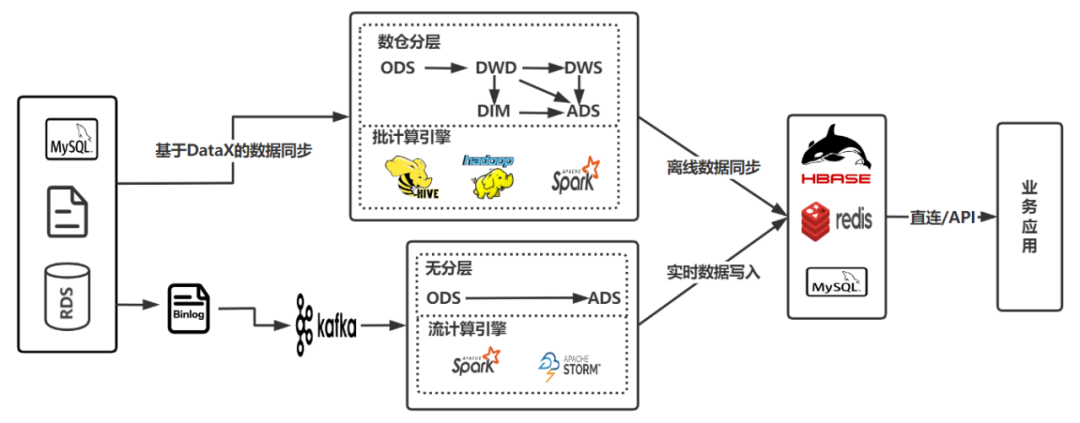 + +原有实时数仓架构是一个典型的 Lambda 架构,上方链路为离线数仓架构,下方链路为实时数据仓架构。鉴于当时离线数仓体系已经非常成熟,使用 Lambda 架构足够支撑实时分析需求,但随着业务的高速发展和数据需求的不断提升,**原有架构凸显出几个问题**: + +- 在实际的流式作业开发中,缺乏对实时数据源的管理,在极端情况下接近于烟囱式接入实时数据流,无法关注数据是否有重复接入,也无法辨别数据是否可以复用。 +- 离线、实时数仓完全割裂,实时数仓没有进行数仓分层,无法像离线数仓按层复用,只能面向业务定制化开发。 +- 数据仓库数据服务于业务平台需要多次中转,且涉及到多个技术组件,ToB 应用亟需引入 OLAP 引擎缓解压力。 +- 计算引擎和存储引擎涉及技术栈多,学习成本和运维难度也很大,无法进行合理有效管理。 + + + +# 新实时数仓架构 + +### **技术选型** + +带着以上的问题,我们希望引入一款成熟的、在业内有大规模落地经验的 OLAP 引擎来帮助我们解决原有架构的痛点。我们希望该 OLAP 引擎不仅要具备传统 OLAP 的优势(即 Data Analytics),还能更好地支持数据服务(Data Serving)场景,比如标签数据需要明细级的查询、实时业务数据需要支持点更新、高并发以及大数据量的复杂 Join 。除此之外,我们希望该 OLAP 引擎可以便捷、低成本的的集成到 Lambda 架构下的离线/实时数仓架构中。**立足于此,我们在技术选型时对比了市面上的几款 OLAP 引擎,如下图所示**: + +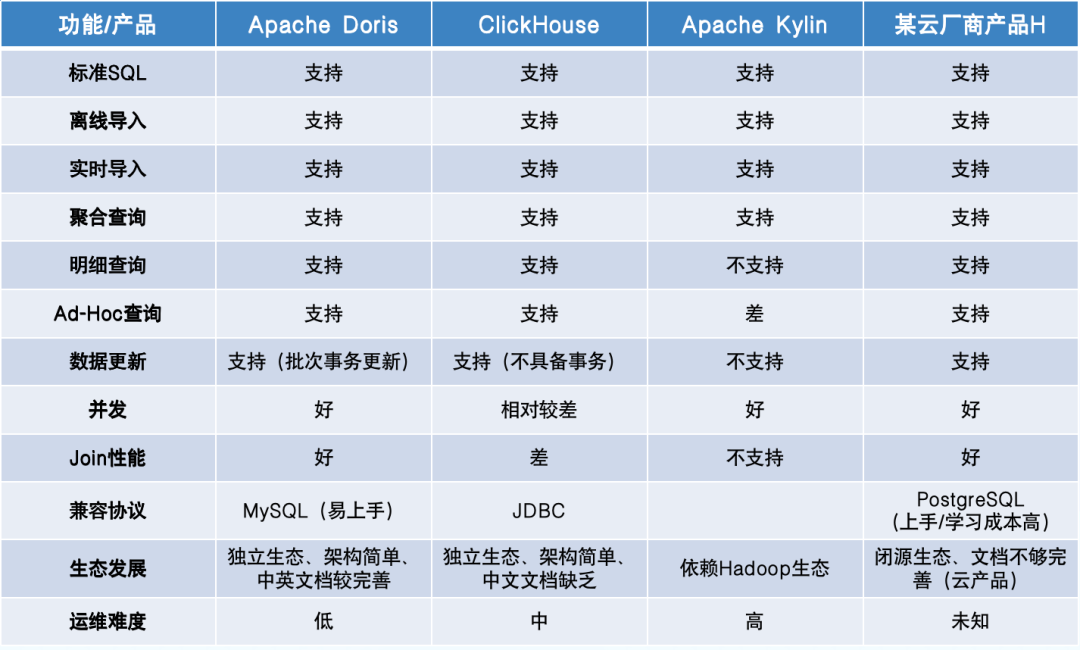 + +根据对选型的要求,**我们发现 Apache Doris 可以很好地满足当前业务场景及诉求,同时也兼顾了低成本的要求,因此决定引入 Doris 进行升级尝试**。 + +### **架构设计** + +我们在 2022 年引入了 Apache Doris ,并基于 Apache Doris 构建了一套比较相对完整的实时数仓架构,如下图所示。 + +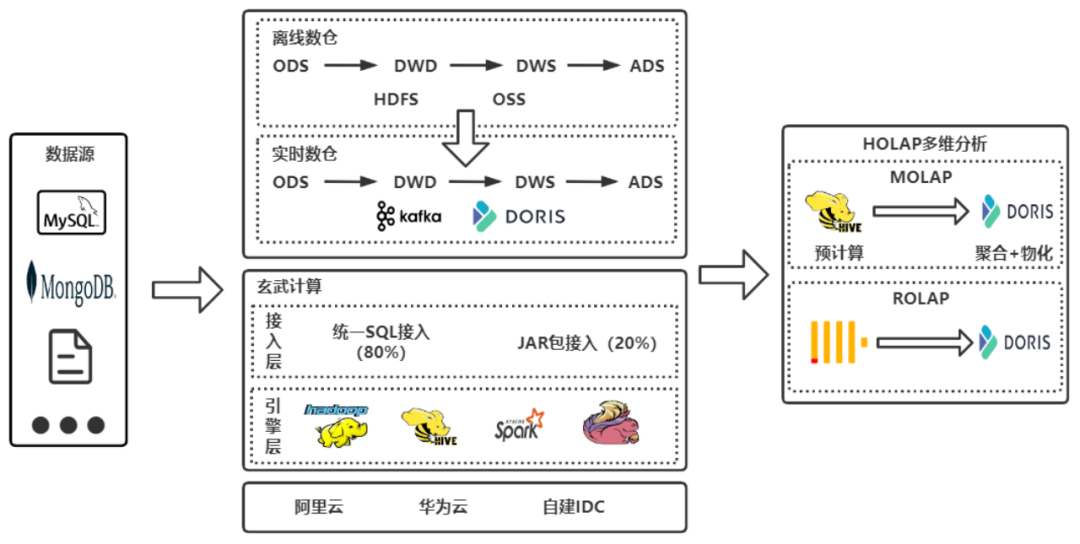 + +总的来说,引入 Doris 后为整体架构带来几大变化: + +- 统一了计算平台(玄武计算),底层引擎支持 Flink、Spark 等组件,接入层支持统一 SQL 和 JAR 包接入。 +- 引入 Doris 后,我们将实时数仓分为 ODS、DWD、DWS、ADS 层,部分中间层实时数据直接使用 Doris 进行存储; +- 构建了基于 Doris 的 HOLAP 多维分析平台,直接服务于业务;简化了原来需要通过 Hive 进行预计算的加工链路,逐步替换使用难度和运维难度相对较高的 ClickHouse; +- 下游应用的数据存储从之前的 MySQL 和 HBase 更换为 Doris,可以在数据集市和大宽表的数据服务场景下直接查询 Doris。 +- 支持混合 IDC(自建和云厂商)。 + +### Overwrite 语义实现 + +Apache Doris 支持原子替换表和分区,我们在计算平台(玄武平台)整合 Doris Spark Connector 时进行了定制,且在 Connector 配置参数上进行扩展、增加了“Overwrite”模式。 + +当 Spark 作业提交后会调用 Doris 的接口,获取表的 Schema 信息和分区信息。 + +- 如果为非分区表:先创建目标表对应的临时表,将数据导入到临时表中,导入后进行原子替换,如导入失败则清理临时表; +- 如果是动态分区表:先创建目标分区对应的临时分区,将数据导入临时分区,导入后进行原子替换,如导入失败则清理临时分区; +- 如果是非动态分区:需要扩展 Doris Spark Connector 参数配置分区表达式,配完成后先创建正式目标分区、再创建其临时分区,将数据导入到临时分区中,导入后进行原子替换,如导入失败则清理临时分区。 + +### 架构收益 + +通过架构升级及二次开发,我们获得了 3 个明显的收益: + +- 构建了规范、完善、计算统一的实时数仓平台 +- 构建了统一混合 OLAP 平台,既支持 MOLAP,又支持 ROLAP,大部分多维分析需求均由该平台实现。 +- 面对大批量数据导入的场景,任务吞入率和成功率提升了 50%。 + + + +# Doris 在标签中台的应用 + +标签中台(也称用户画像平台)是斗鱼进行精准运营的重要平台之一,承担了各业务线人群圈选、规则匹配、A/B 实验、活动投放等需求。接下来看下 Doris 在标签中台是如何应用的。 + +### 原标签中台架构 + +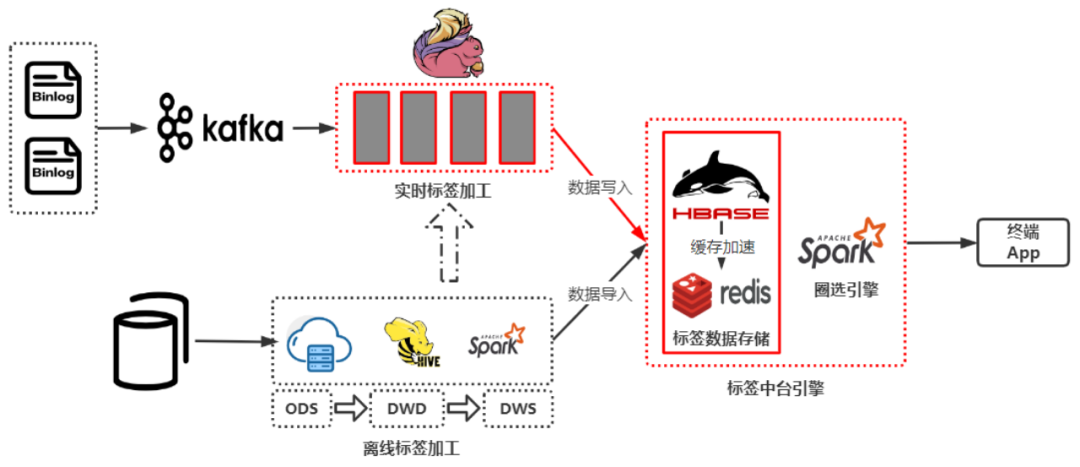 + +上图为斗鱼原来的标签中台架构,离线标签在数仓中加工完成后合入宽表,将最终数据写入 HBase 中,实时标签使用 Flink 加工,加工完直接写入到 HBase 中。 + +终端 APP 在使用标签中台时,主要解决两种业务需求: + +- 人群圈选,即通过标签和规则找到符合条件的人。 +- 规则匹配,即当有一个用户,找出该用户在指定的业务场景下符合哪些已配置的规则,也可以理解是“人群圈选“的逆方向。 + +在应对这两种场需求中,原标签中台架构出现了两个问题: + +- 实时标签链路:Flink 计算长周期实时指标时稳定性较差且耗费资源较高,任务挂掉之后由于数据周期较长,导致 Checkpoint 恢复很慢; +- 人群圈选:Spark 人群圈选效率较低,特别是在实时标签的时效性上。 + +### 新标签中台架构 + +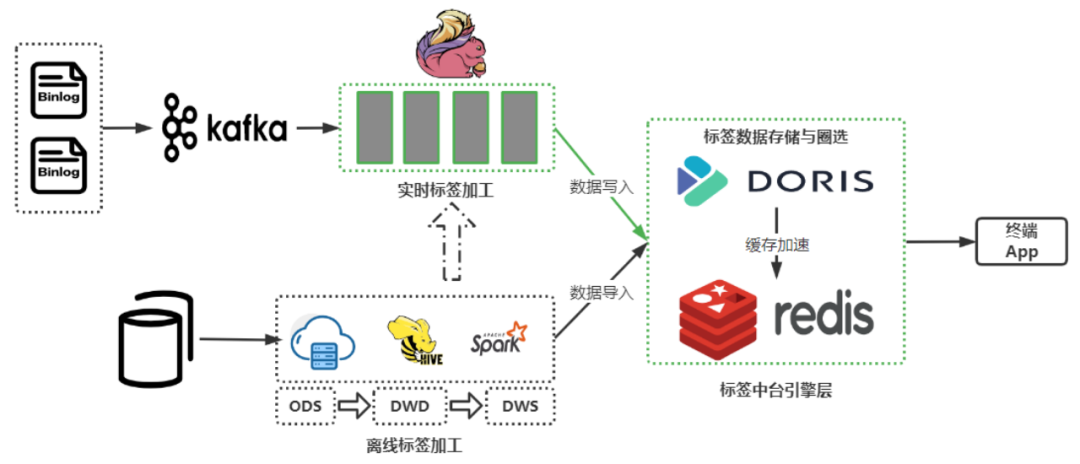 + +引入 Apache Doris 之后,我们对标签中台架构的进行了改进,主要改进集中在实时链路和标签数据存储这两个部分: + +- 实时标签链路:仍然是通过实时数据源到 Kafka 中,通过 Flink 进行实时加工;不同的是,我们将一部分加工逻辑迁移到 Doris 中进行计算,长周期实时指标的计算从单一的 Flink 计算转移到了 Flink + Doris 中进行; +- 标签数据存储:从 HBase 改成了 Doris,利用 Doris 聚合模型的部分更新特性,将离线标签和实时标签加工完之后直接写入到 Doris 中。 + +#### **1. 离线/实时标签混合圈人** + +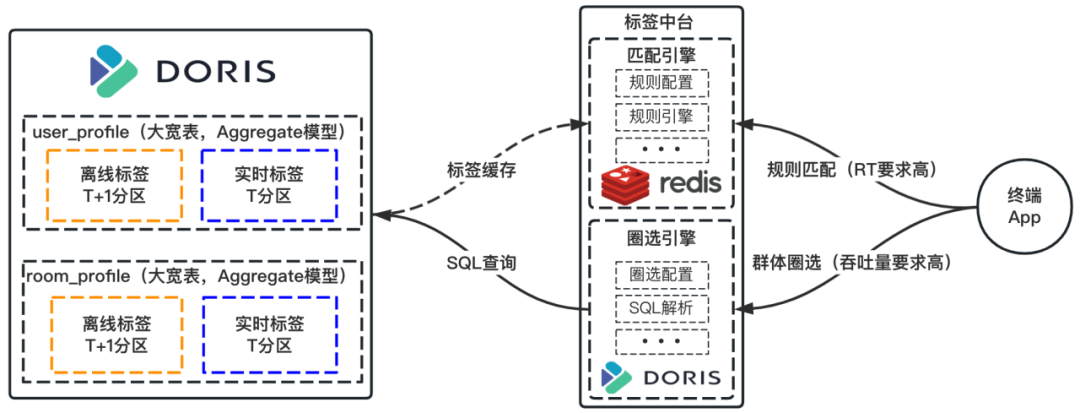 + +- 简化存储:原存储在 HBase 中的大宽表,改为在 Doris 中分区存储,其中离线标签 T+1 更新,实时标签 T 更新、T+1 采用离线数据覆盖矫正。 +- 查询简化:**面对人群圈选场景**,无需利用 Spark 引擎,可直接在标签中台查询服务层,将圈选规则配置解析成 SQL 在 Doris 中执行、并获得最终的人群,大大提高了人群圈选的效率。**面对规则匹配场景**,使用 Redis 缓存 Doris 中的热点数据,以降低响应时间。 + +#### **2. 长周期实时标签计算原链路** + +长周期实时标签:计算实时标签时所需的数据周期较长,部分标签还需要采用历史数据(离线)合并实时数据流一起进行计算的场景。 + +**使用前:** + +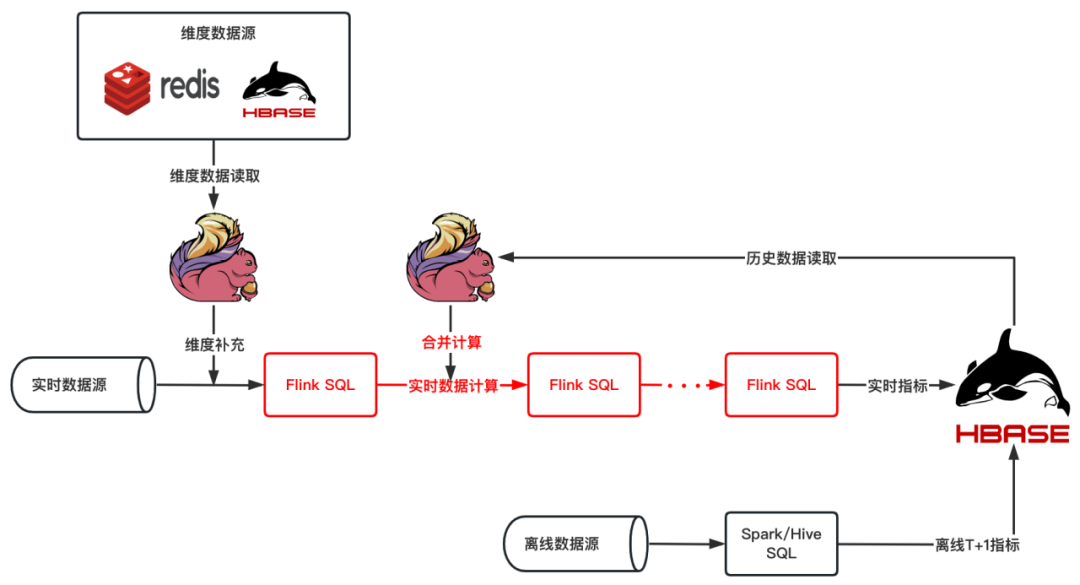 + +从原来的计算链路中可知,计算长周期的实时标签时会涉及到维度补充、历史数据 Merge,在经过几步加工最终将数据写入到 HBase 中。 + +在实际使用中发现,在这个过程中 Merge 离线聚合的数据会使链路变得很复杂,往往一个实时标签需要多个任务参与才能完成计算;另外聚合逻辑复杂的实时标签一般需要多次聚合计算,任意一个中间聚合资源分配不够或者不合理,都有可能出现反压或资源浪费的问题,从而使整个任务调试起来特别困难,同时链路过长运维管理也很麻烦,稳定性也比较低。 + +**使用后:** + +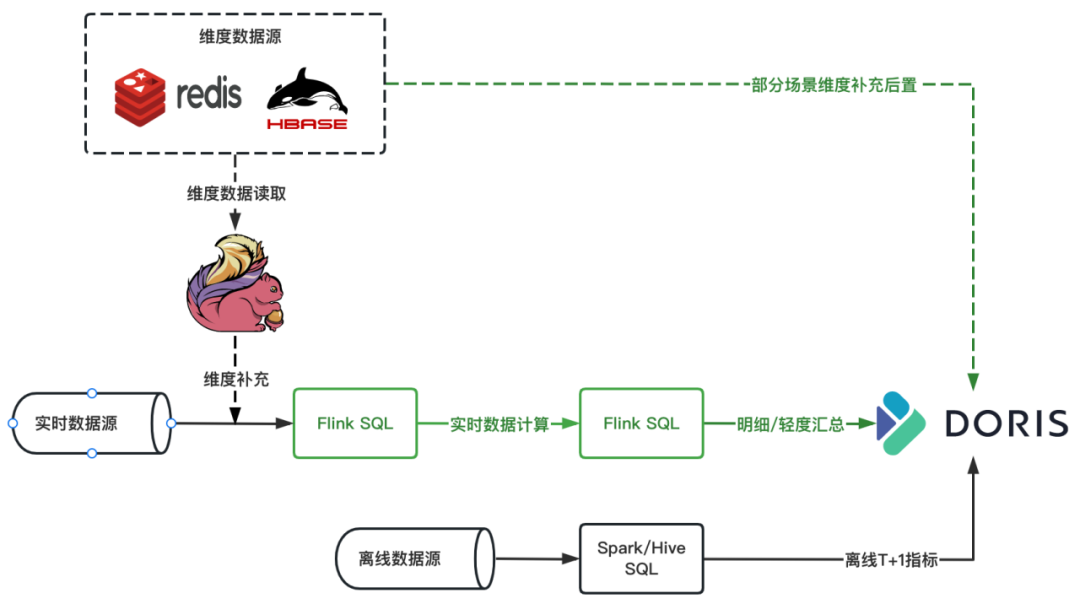 + +我们在长周期指标计算实时链路中加入了 Apache Doris,在 Flink 中只做维度补充和轻度加工汇总,只关注短的实时数据流,对于需要 Merge 的离线数据,Merge 的计算逻辑转移到 Doris 中进行计算,另外 Doris 中的轻度汇总/明细数据有助于问题排查,同时任务稳定性也能提升。 + +### 使用收益 + +目前标签中台底层有近 4 亿+条用户标签,每个用户标签 300+,已有 1W+ 用户规则人群,每天定时更新的人群数量达到 5K+。标签中台引入 Apache Doris 之后,**单个人群平均圈选时间实现了分钟级到秒级的跨越**,实时标签任务稳定性有所提高,实时标签任务的产出时间相较于之前约有 **40% 的提升**,资源使用成本大大降低。 + + + + + +# Doris 在多维数据分析平台的应用 + +除以上所述应用及收益之外,Apache Doris 也助力内部多维数据分析平台——斗鱼 360 取得了较大的发展,受益于 Apache Doris 的 Rollup、物化视图以及向量化执行引擎,使原来需要预计算的场景可以直接导入明细数据到 Doris 中,简化了业务数据开发流程,提升了分析效率;Doris 兼容 MySQL 协议,并具有独立简单的分布式架构,使得业务开发人员入门使用也更容易,缩短了业务开发周期,有效降低了开发成本;同时我们原来基于 ClickHouse 的查询目前也全部切换到了 Doris 中进行。 + +目前我们用于多维分析场景的 Doris 集群共有两个,节点规模约 120 个,存储数据量达 90~100 TB,每天增量写入到 Doris 的数据约 900GB,其中查询 QPS 在 120 左右,Apache Doris 应对起来毫不费力,轻松自如。 + +*因文章篇幅限制,该部分应用不再赘述,后续有机会与大家进行详细分享。* + + + +# 未来展望 + +未来随着 Apache Doris 在斗鱼更广泛业务场景的落地,我们将在可视化运维、问题快速定位排查等方面进行更多实践和深耕。我们关注到, Apache Doris 1.2.0 版本已经对 Multi Catalog 功能进行了支持,我们也计划对其进行探索、解锁更多使用场景,同时也期待即将发布 Apache Doris 2.x 版本的行列混存功能,更好的支持 Data Serving 场景。 \ No newline at end of file diff --git a/static/images/Douyu_1.png b/static/images/Douyu_1.png new file mode 100644 index 00000000000..e718aebf008 Binary files /dev/null and b/static/images/Douyu_1.png differ diff --git a/static/images/Douyu_2.png b/static/images/Douyu_2.png new file mode 100644 index 00000000000..adf3055df40 Binary files /dev/null and b/static/images/Douyu_2.png differ diff --git a/static/images/Douyu_3.png b/static/images/Douyu_3.png new file mode 100644 index 00000000000..5340a41c01e Binary files /dev/null and b/static/images/Douyu_3.png differ diff --git a/static/images/Douyu_4.png b/static/images/Douyu_4.png new file mode 100644 index 00000000000..84fea71476a Binary files /dev/null and b/static/images/Douyu_4.png differ diff --git a/static/images/Douyu_5.png b/static/images/Douyu_5.png new file mode 100644 index 00000000000..d5d16b63dcf Binary files /dev/null and b/static/images/Douyu_5.png differ diff --git a/static/images/Douyu_6.png b/static/images/Douyu_6.png new file mode 100644 index 00000000000..54e95909dce Binary files /dev/null and b/static/images/Douyu_6.png differ --------------------------------------------------------------------- To unsubscribe, e-mail: commits-unsubscr...@doris.apache.org For additional commands, e-mail: commits-h...@doris.apache.org